Zero Shot Learning
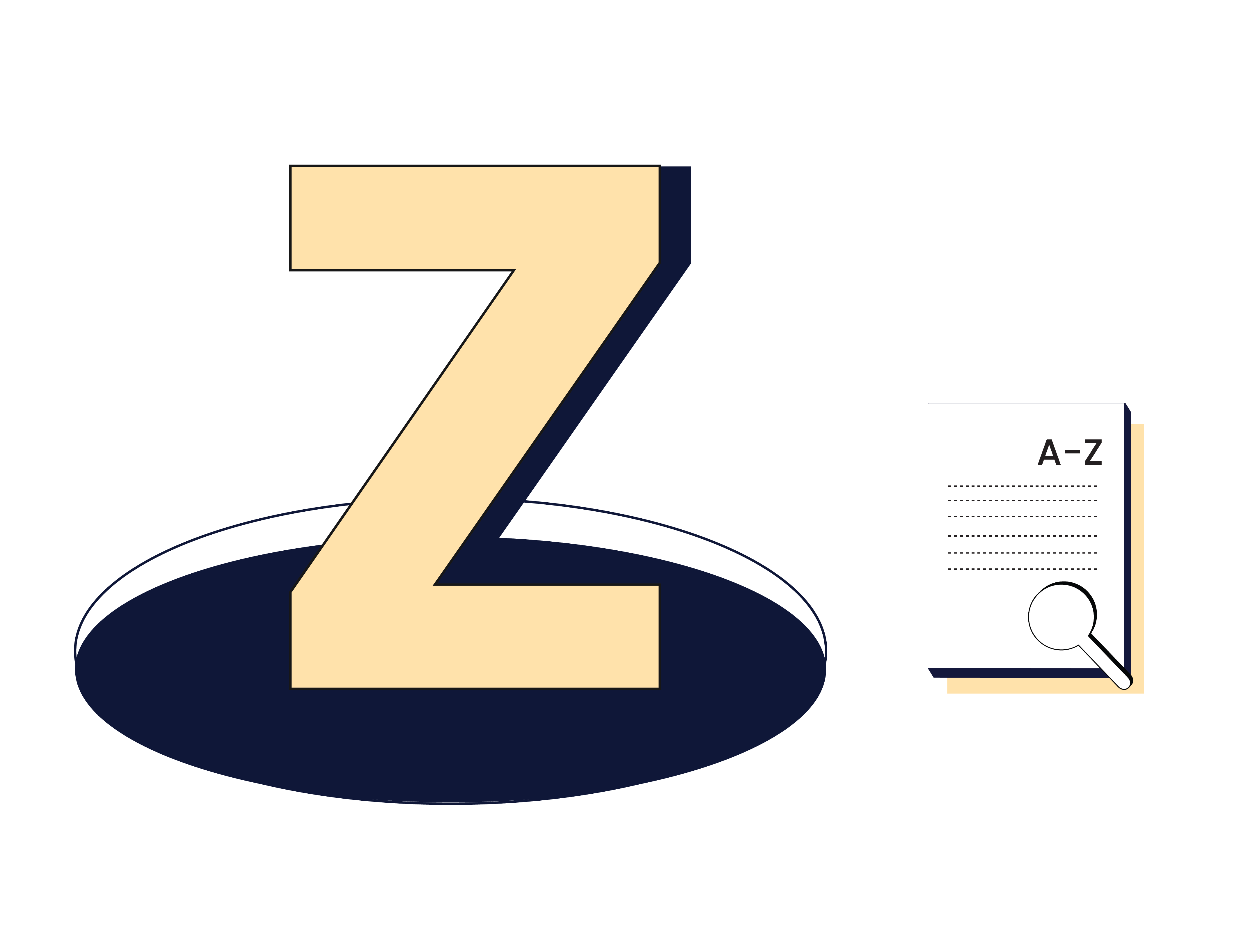
What is Zero Shot Learning?
Zero-shot learning serves as the cornerstone for the development of generative AI methods.
Zero-shot learning, along with one-shot learning, constitutes a methodology enabling generative AI models to grasp and categorize novel instances despite having access to only a restricted volume of training data. This concept pertains to the model’s capability to classify novel, unseen instances belonging to categories that were absent from the training dataset.
Examples of zero-shot learning:
- Image Recognition and captioning: For example, a model that has been trained on images of dogs and cats could potentially identify an image of a tiger by drawing parallels with analogous attributes present in its training dataset.
- Natural Language Processing (NLP): Zero-shot learning facilitates comprehension and generation of previously untrained text. Its utility is pronounced in tasks like translation, sentiment analysis, and text generation.
- Medical Diagnosis: Zero-shot learning allows the model to recognize illnesses or ailments that it lacks training data for.
- Object detection: Zero-shot learning enables the identification of objects in images or videos that the model isn’t trained to identify. For instance, a model focused on cars and trucks could potentially spot a bicycle within an image, despite lacking bicycle-specific training.
- Computational Biology: It helps forecast traits of unobserved biological entities. For instance, it can predict the characteristics of a novel virus strain using its genetic sequence and supplemental data about analogous viruses.
Read more: Zero Shot Learning: A complete guide
Related Resources
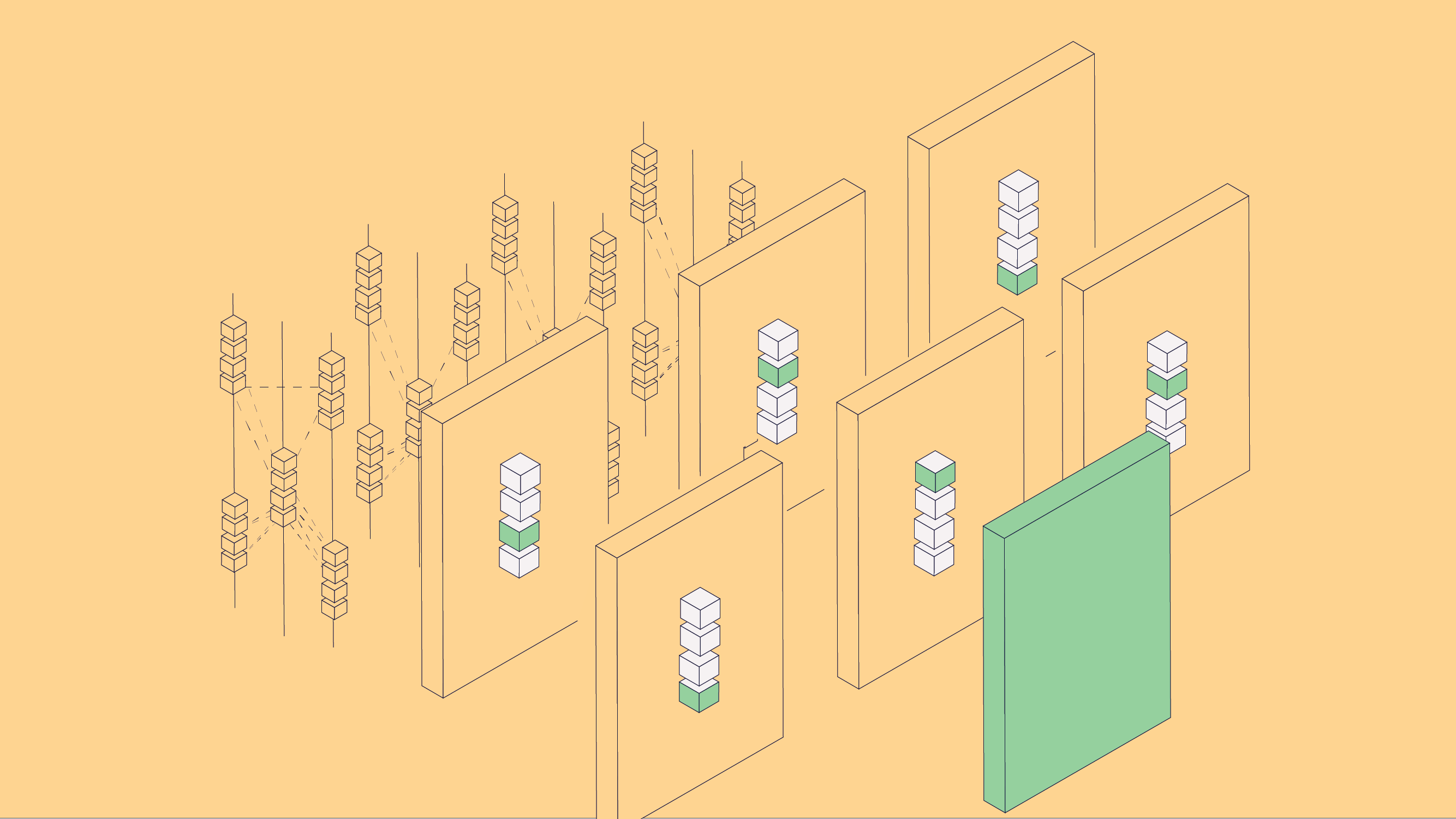
Zero Shot Learning: A complete guide
In the realm of the big screen, there’s a man who needs no introduction. A man of resourcefulness, a man of ingenuity, a man who could turn a paperclip into a key to conquer the most impossible of missions. His name? Ethan Hunt. He is the embodiment of the idea that necessity is the mother […]
Read More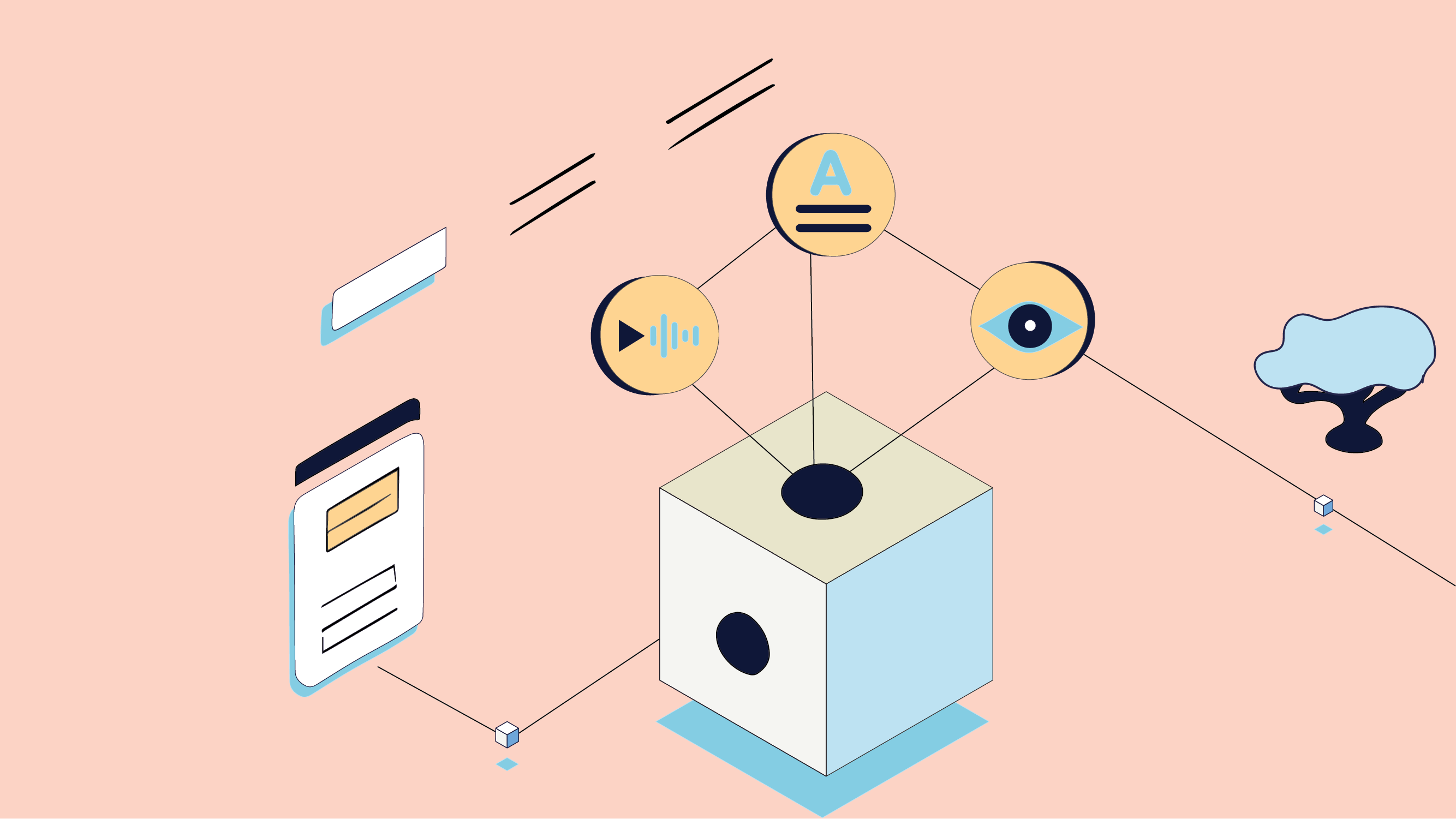
Multimodal Learning In AI: Introduction, Current Trends, and Future
As a conductor stands poised on the podium, baton aloft, they survey the orchestra before them. Each musician holds a different instrument, a unique voice in the grand symphony they are about to perform. Violins, their strings humming with anticipation, are primed to sing the melody. Cellos stand ready to resonate with harmony, the percussion […]
Read More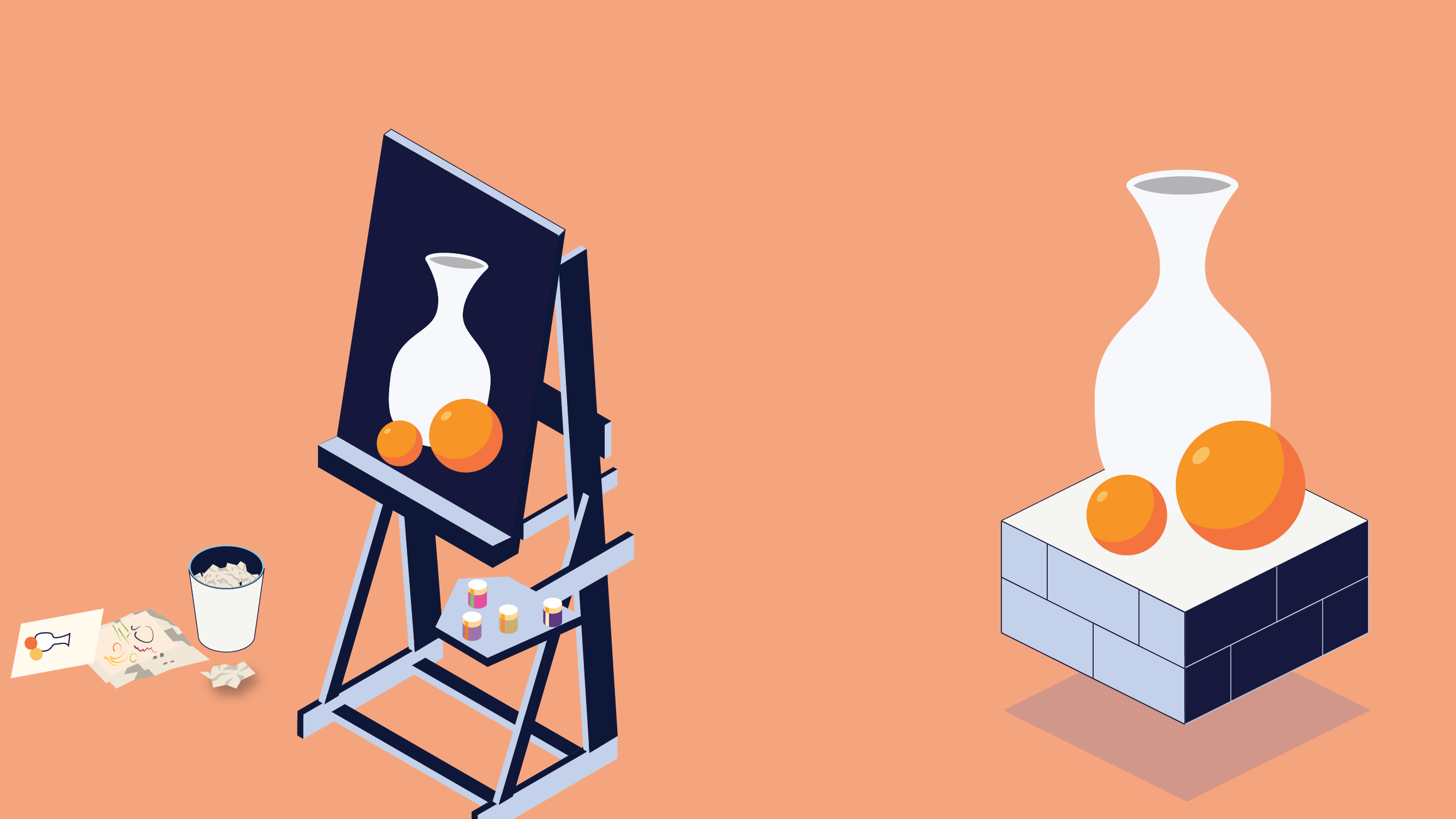
Mastering Generative AI: A comprehensive guide
The year was 2018. Art enthusiasts, collectors, and critics from around the world gathered at Christie’s, one of the most prestigious auction houses. The spotlight was on a unique portrait titled “Edmond de Belamy.” At first glance, it bore the hallmarks of classical artistry: a mysterious figure, blurred features reminiscent of an old master’s touch, […]
Read MoreStay updated on the latest and greatest at Scribble Data
Sign up to our newsletter and get exclusive access to our launches and updates