Transfer Learning
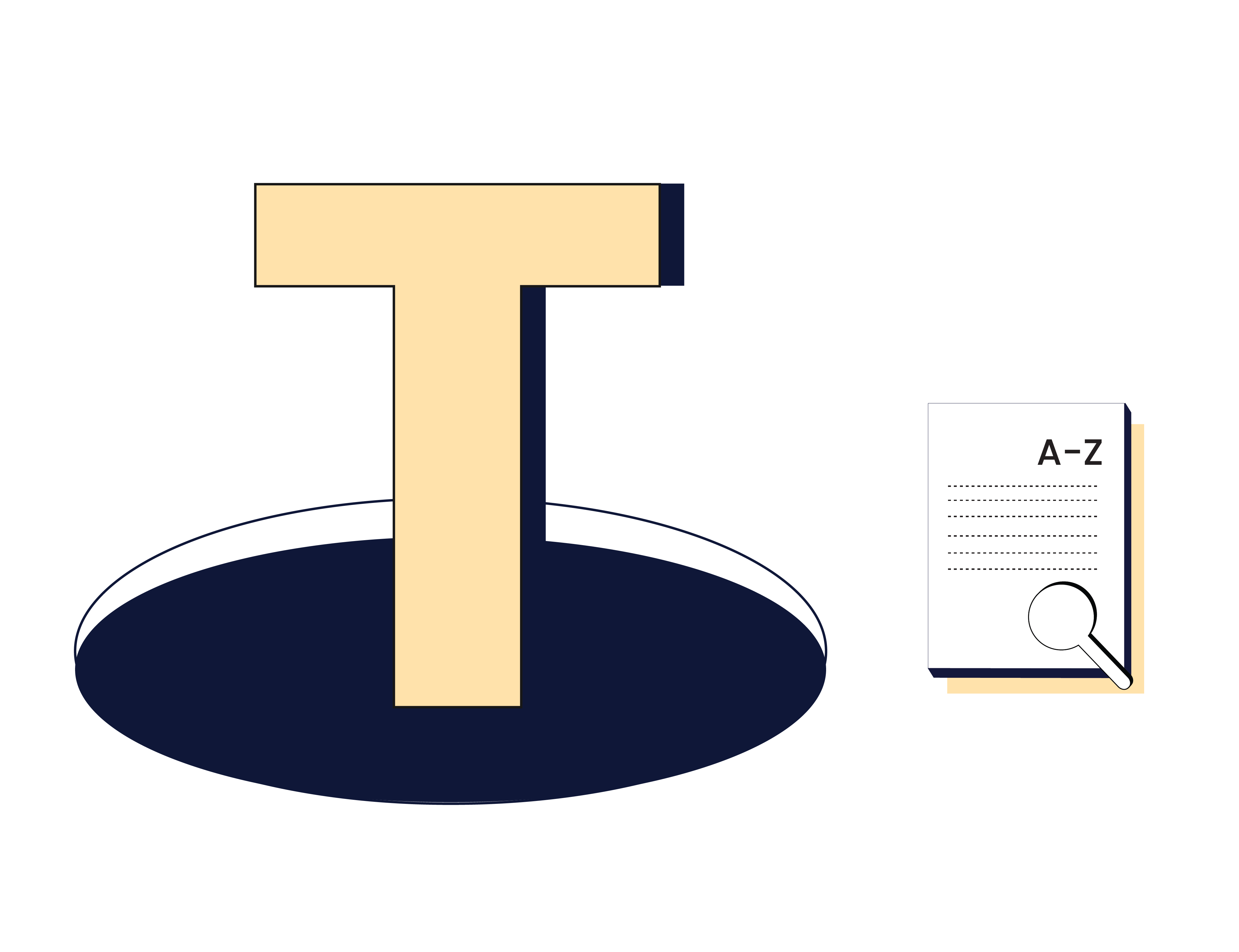
What is Transfer Learning?
Transfer learning in AI is a technique that allows a model to leverage knowledge from one domain and apply it to another domain. It is a powerful tool that helps to overcome the limitations of traditional machine learning approaches, where each model is trained from scratch on a specific dataset. With transfer learning, models can be pre-trained on large datasets, such as ImageNet, and then fine-tuned on smaller, domain-specific datasets. This approach not only saves computational resources but also improves the performance of the model in the target domain.
One of the key benefits of transfer learning in AI is its ability to generalize knowledge across different tasks. By learning from a large, diverse dataset, a model can capture meaningful patterns and features that are relevant to multiple domains. This enables the model to transfer its learned knowledge to new tasks, even if the new tasks have limited training data. For example, a model pre-trained on image classification can be used for object detection or image segmentation tasks with only a small amount of additional training data.
Transfer learning also plays a crucial role in solving the problem of insufficient training data. In many real-world scenarios, obtaining labeled data for training a model can be expensive or time-consuming. Transfer learning allows us to leverage existing pre-trained models and adapt them to our specific needs with limited labeled data. This reduces the dependence on large-scale labeled datasets and accelerates the development of new AI applications.
In conclusion, transfer learning in AI is an effective technique that enables models to leverage knowledge from one domain and apply it to another. It improves the performance of models by generalizing knowledge across different tasks and helps overcome the limitations of insufficient training data. By pre-training models on large datasets and fine-tuning them on smaller, domain-specific datasets, transfer learning saves computational resources and accelerates the development of AI applications.
Related Article: https://www.scribbledata.io/transfer-learning-in-ai-a-complete-guide/
Related Resources
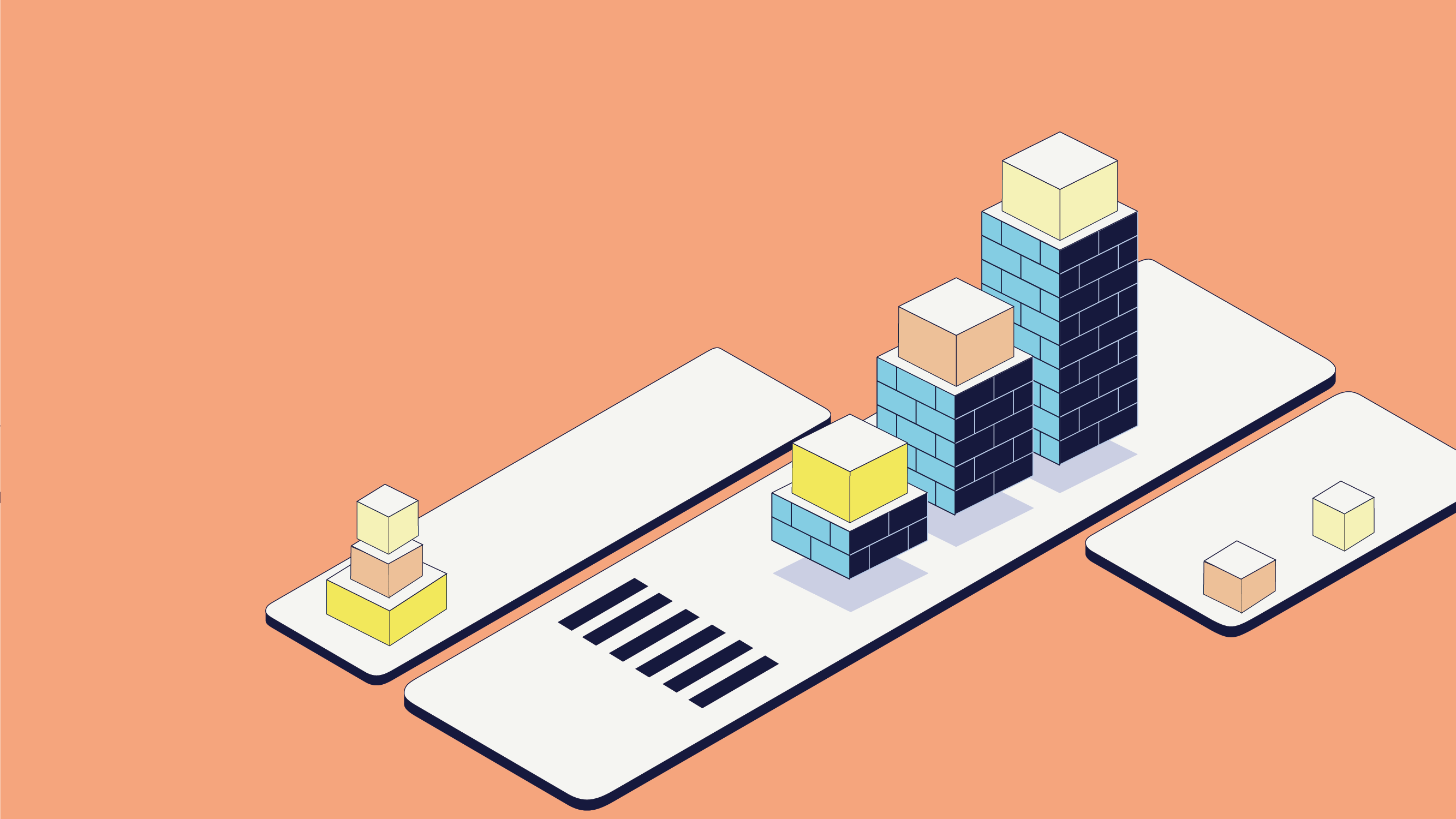
Transfer learning in AI: A complete guide
Picture yourself as a culinary maestro. You have dedicated countless hours in the kitchen, mastering the nuances of French cuisine, perfecting the art of sourdough, and orchestrating symphonies of flavor in a well-risen chocolate soufflé. Each culinary expedition has bestowed upon you a wealth of knowledge—harmonizing tastes, kneading the dough with finesse, and deftly tempering […]
Read More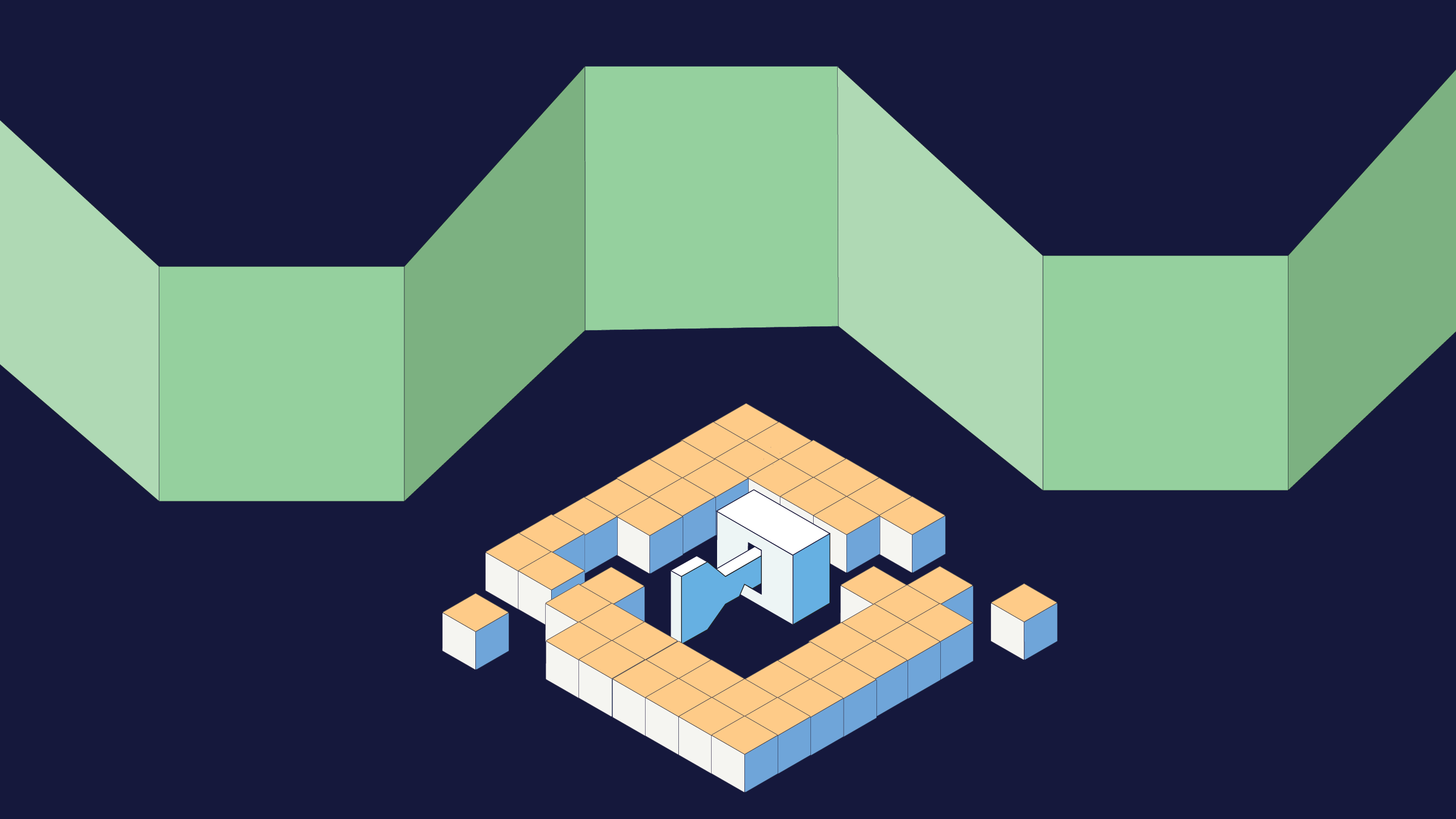
Generative AI: A Technical Deep Dive into Security and Privacy Concerns
In a tale as old as time, King Midas yearned for a touch that could metamorphose all to gold. His wish was granted, and the world around him shimmered with the allure of endless wealth. Every object he grazed turned to gold, dazzling yet cold to the touch. The ecstasy of boundless power was intoxicating, […]
Read More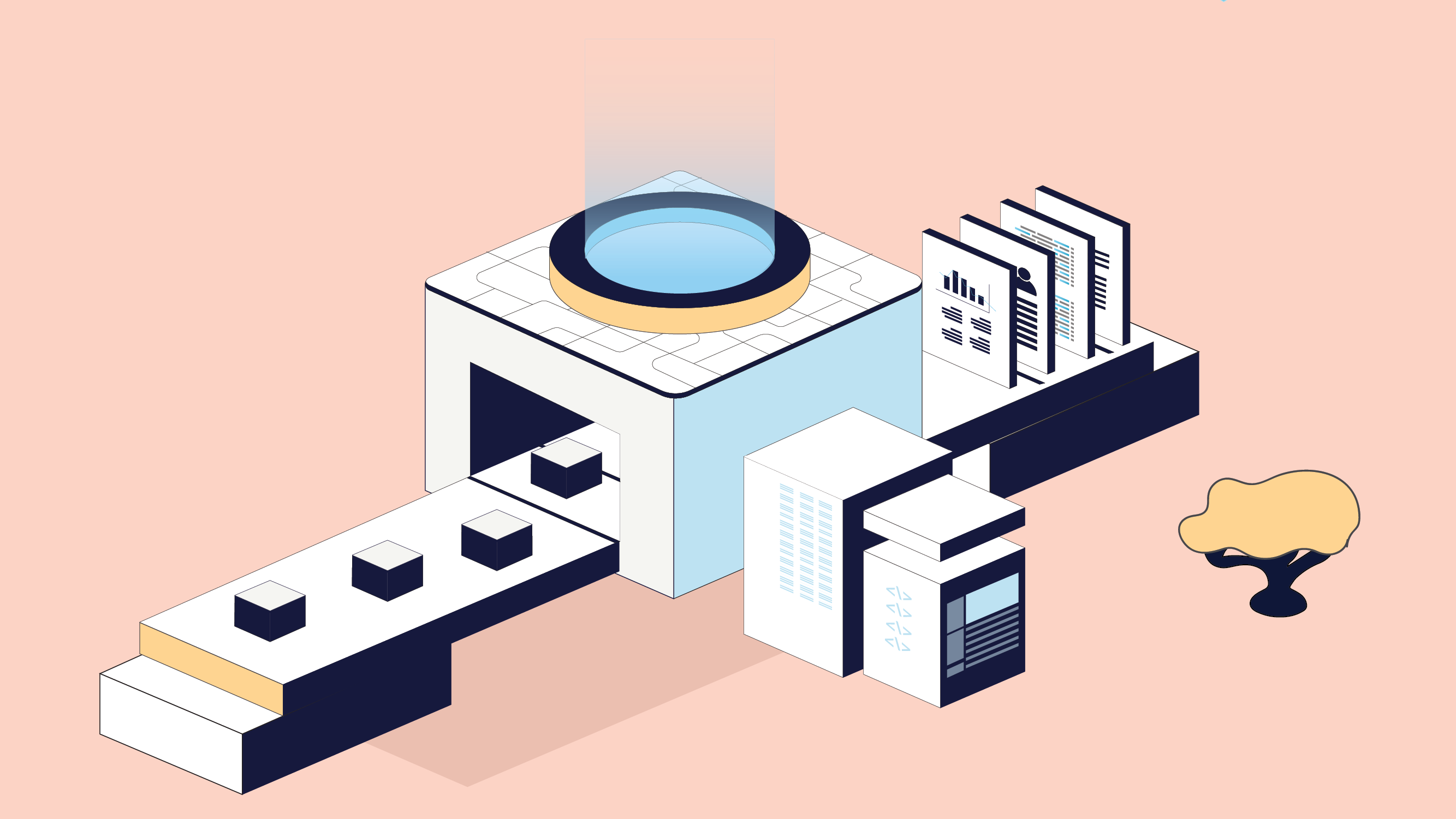
From Data to Decisions: How Generative AI is Transforming Enterprise Analytics
It’s the 24th century aboard the Starship Enterprise. Captain Jean-Luc Picard, in need of a break from the rigors of interstellar diplomacy, steps into the Holodeck. This isn’t just any room; it’s a technological marvel, a space where any scenario can be simulated, any world, any reality can come to life. Picard chooses a 1940s […]
Read MoreStay updated on the latest and greatest at Scribble Data
Sign up to our newsletter and get exclusive access to our launches and updates