Synthetic Data
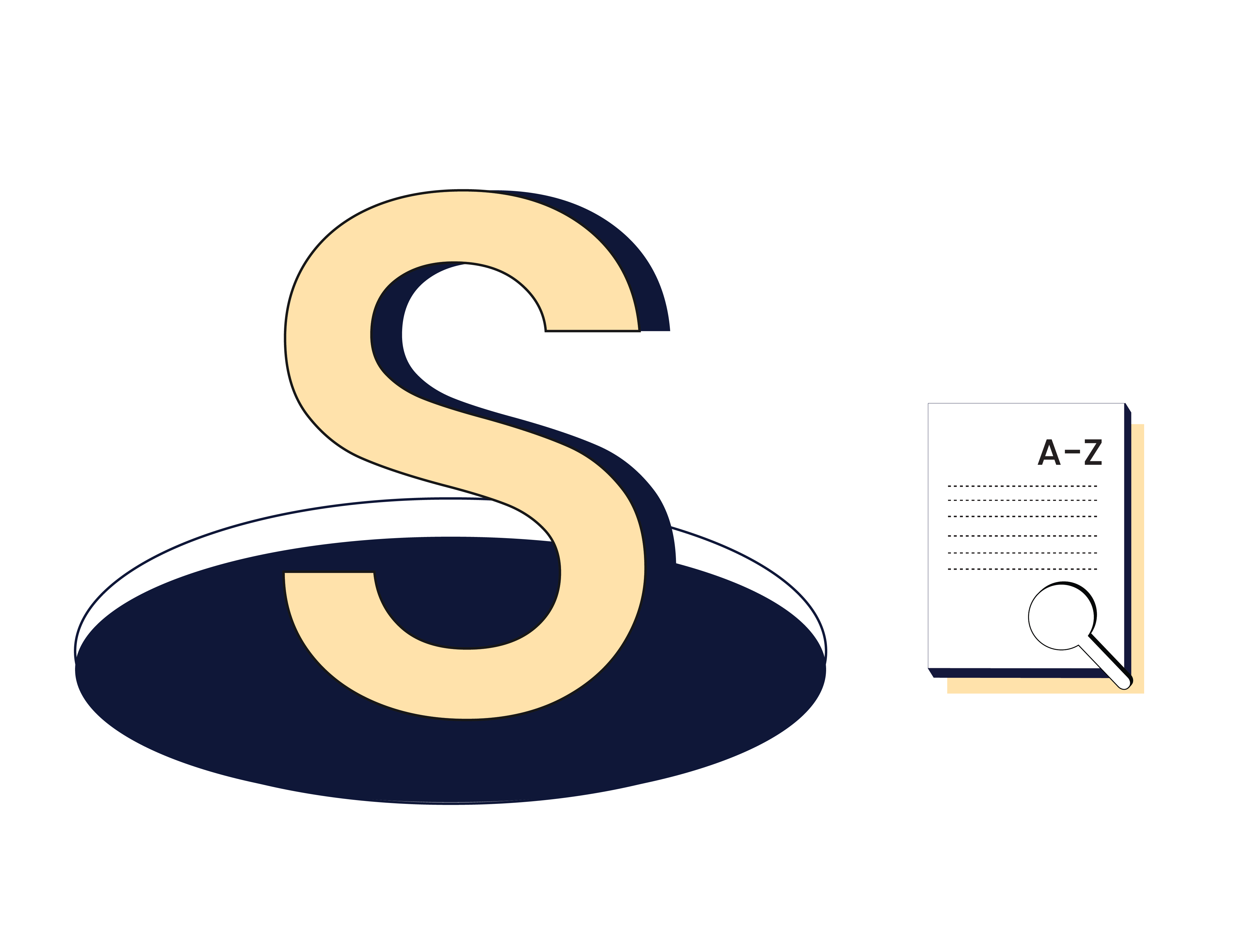
What is synthetic data in machine learning?
Synthetic data is information that is artificially generated, and not derived from real-world occurrences. Often crafted through algorithms, it validates mathematical models and trains machine learning models. Data produced by computer simulations can also qualify as synthetic data.
Applications of Synthetic Data:
- AI/ML Training and Development: Synthetic data enables us to create extensive data customized to our requirements, enhancing model robustness, accuracy, and generalizability.
- Test Data Management: It enables crafting superior, varied, and representative test and validation data, enhancing testing efficiency, accelerating time-to-market, and lowering conventional test data management expenses.
- Data Analytics and Visualization: Synthetic data is a potent asset for data analytics and visualization. It crafts application-specific datasets, enhancing analysis precision and visualization quality. It facilitates modeling intricate situations, showcasing trends, patterns, and insightful data exploration, all while upholding privacy and security.
- Enterprise Data Sharing: Synthetic data enables secure data sharing and collaboration among enterprises. It functions like a shield, permitting work with data while preserving its confidentiality. This empowers businesses to collaborate on data-driven projects, share insights, and foster innovation without endangering data privacy.
- Domain-specific use cases: Tailoring synthetic data to replicate target domain characteristics enhances successful AI system design and testing. This yields more precise models, predictions, and superior outcomes across industries.
Read More: Synthetic Data in Machine Learning: Introduction, Applications, and Future
Related Resources
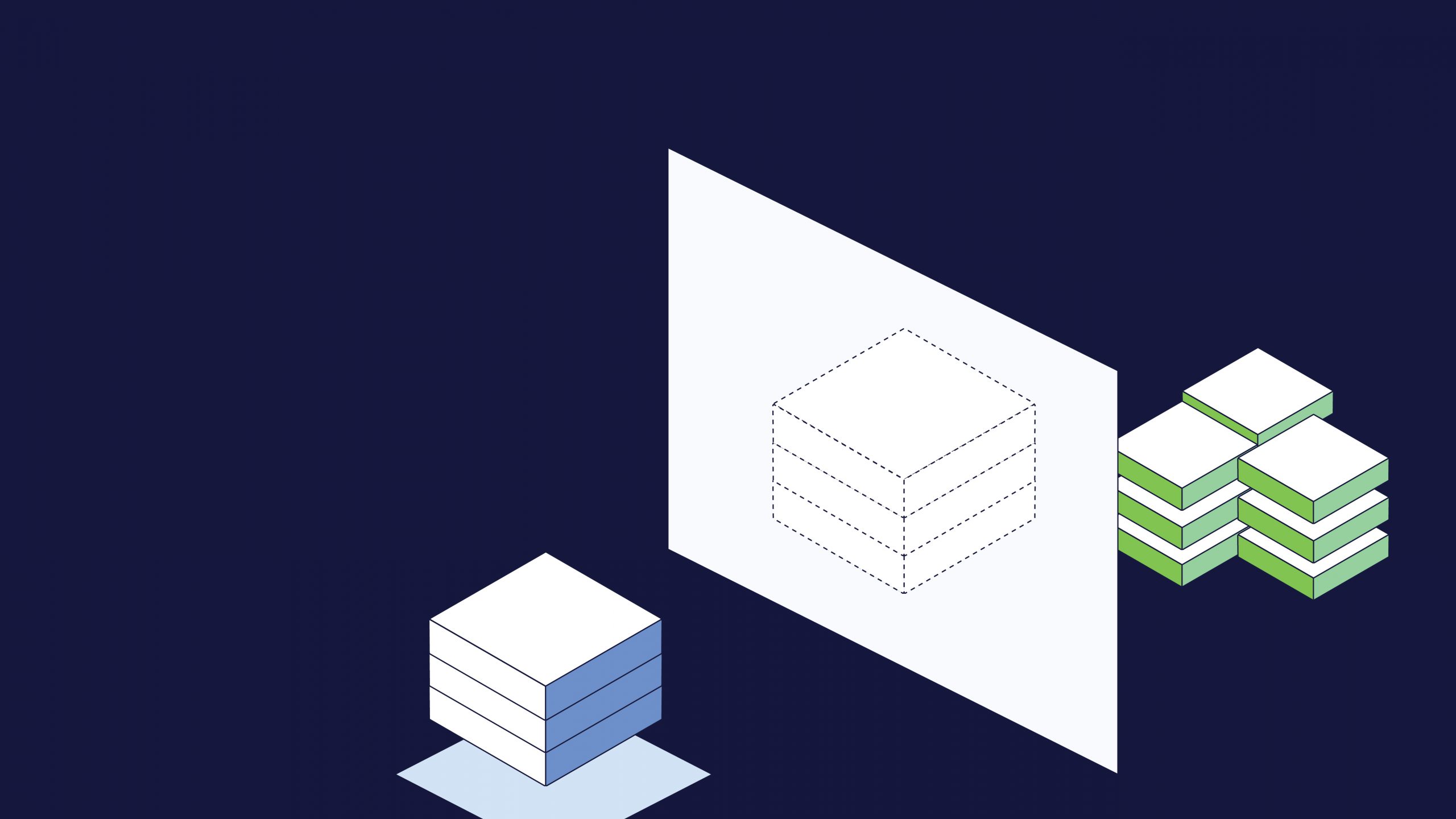
Synthetic Data in Machine Learning: Introduction, Applications, and Future
Picture this: You’re in the world of “Inception,” Christopher Nolan’s cinematic masterpiece. Dream architects are crafting intricate labyrinths within dreams, creating realities so convincing that the dreamer can’t tell they’re asleep. They are bending the fabric of the dream, shaping it to their will, whether it’s a heart-pounding chase through a bustling market or a […]
Read More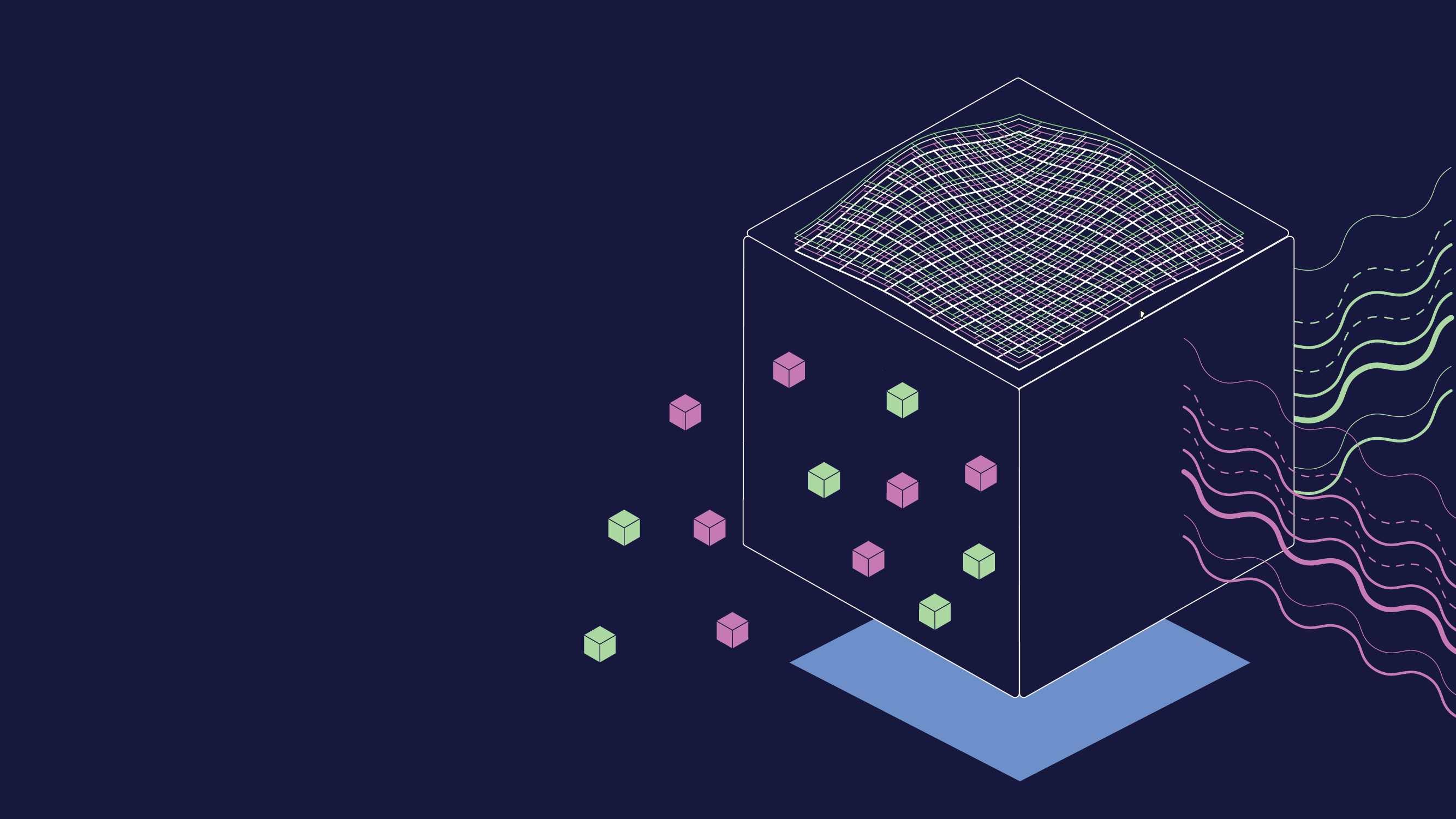
Data Fabric: Unraveling the Future of Integrated Data Management
Scene 1: Picture waking up to the soft strumming of the acoustic guitar on Bon Iver’s “Holocene”, a song recommendation from Spotify based on your recent obsession with indie folk. Scene 2: As you sip your morning coffee, you scroll through your Amazon app, noticing a recommendation for a book on “Modern Folklore and Music.” […]
Read More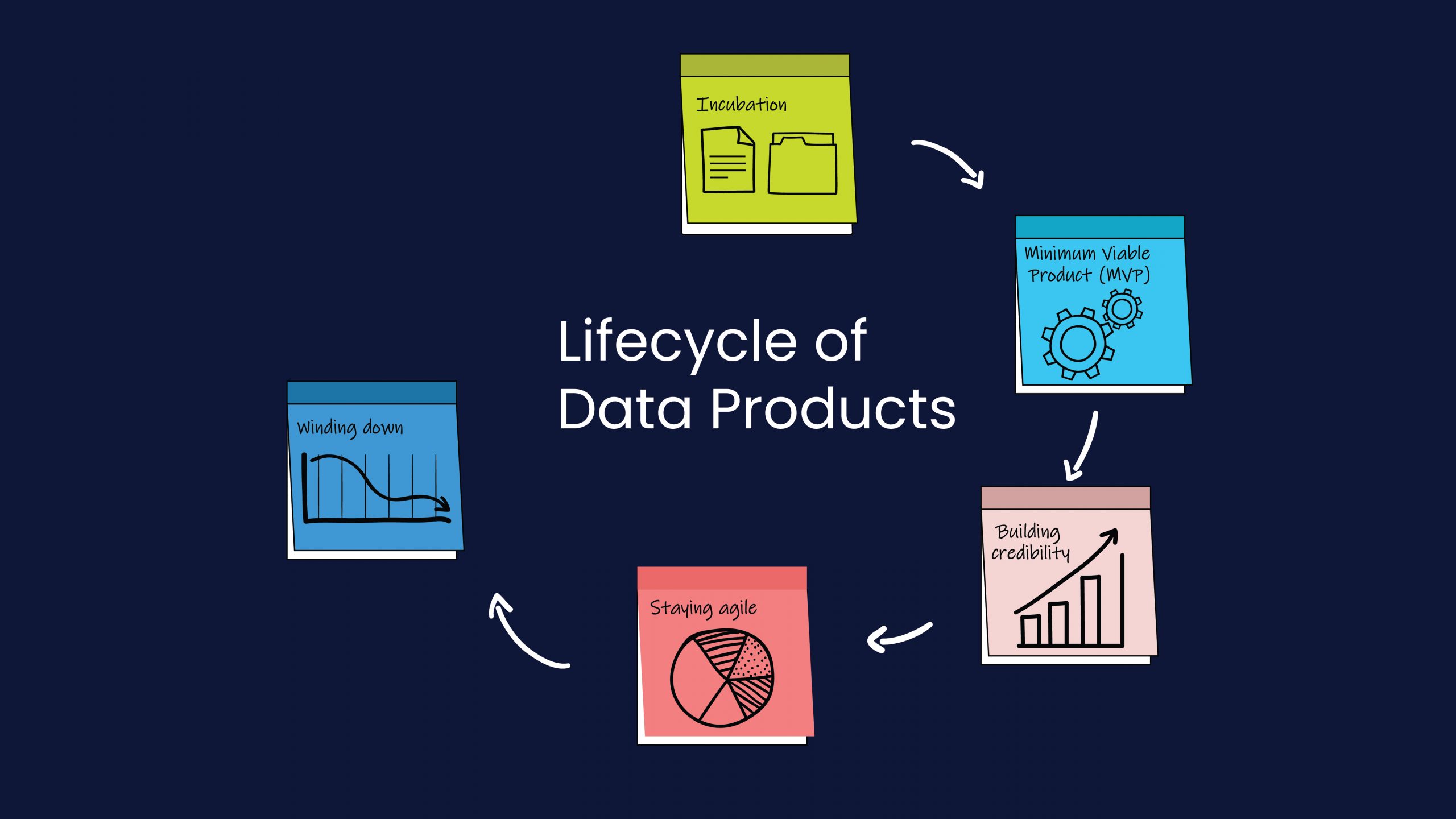
Data Product Lifecycle: Evolution and Best Practices
Data products have exploded in popularity over the last few years. As an industry, we are where the automobile industry was around the turn of the 20th century. We are slowly transitioning from building hand-crafted, exclusive products for Big Tech customers to widespread commoditization. Soon, efficiency, maintenance, standards, and assembly lines are going to be […]
Read MoreStay updated on the latest and greatest at Scribble Data
Sign up to our newsletter and get exclusive access to our launches and updates