Batch Normalization
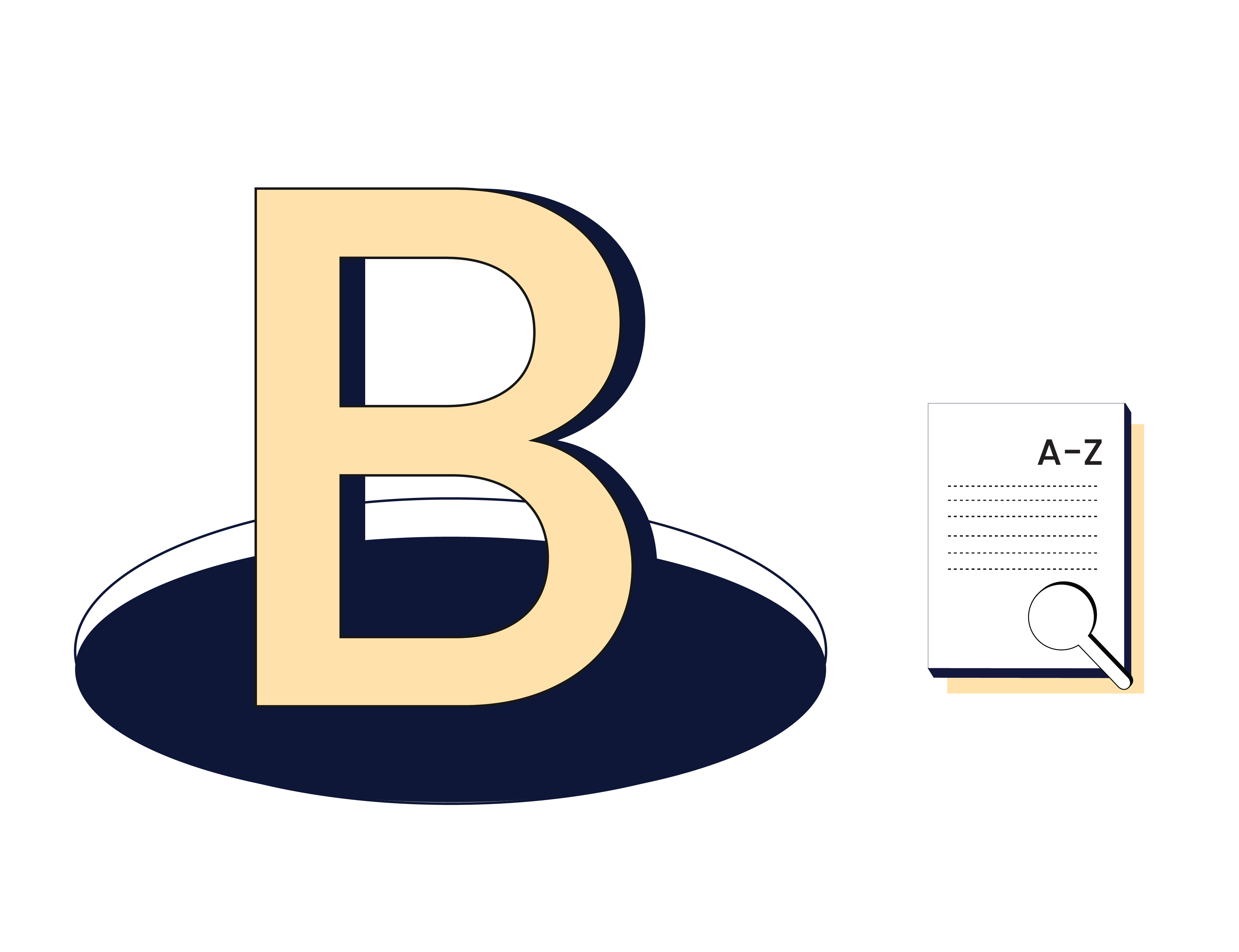
What is Batch Normalization?
Batch normalization is a technique for improving the performance and stability of neural networks. It is commonly used in deep learning, where it has been shown to accelerate the training of deep neural networks and improve their ability to generalize to new data.
Batch normalization works by normalizing the activations of the neurons in a network across a batch of training examples. It does this by subtracting the mean activation of the batch and dividing it by the standard deviation so that the resulting activations have a mean of zero and a standard deviation of one. It stabilizes the distribution of activations and reduces the covariate shift that can occur during training.
In addition to improving the performance and stability of neural networks, batch normalization can also reduce the need for careful weight initialization and make it easier to use higher learning rates. These benefits make it a popular choice for practitioners working with deep learning models.
Related Resources
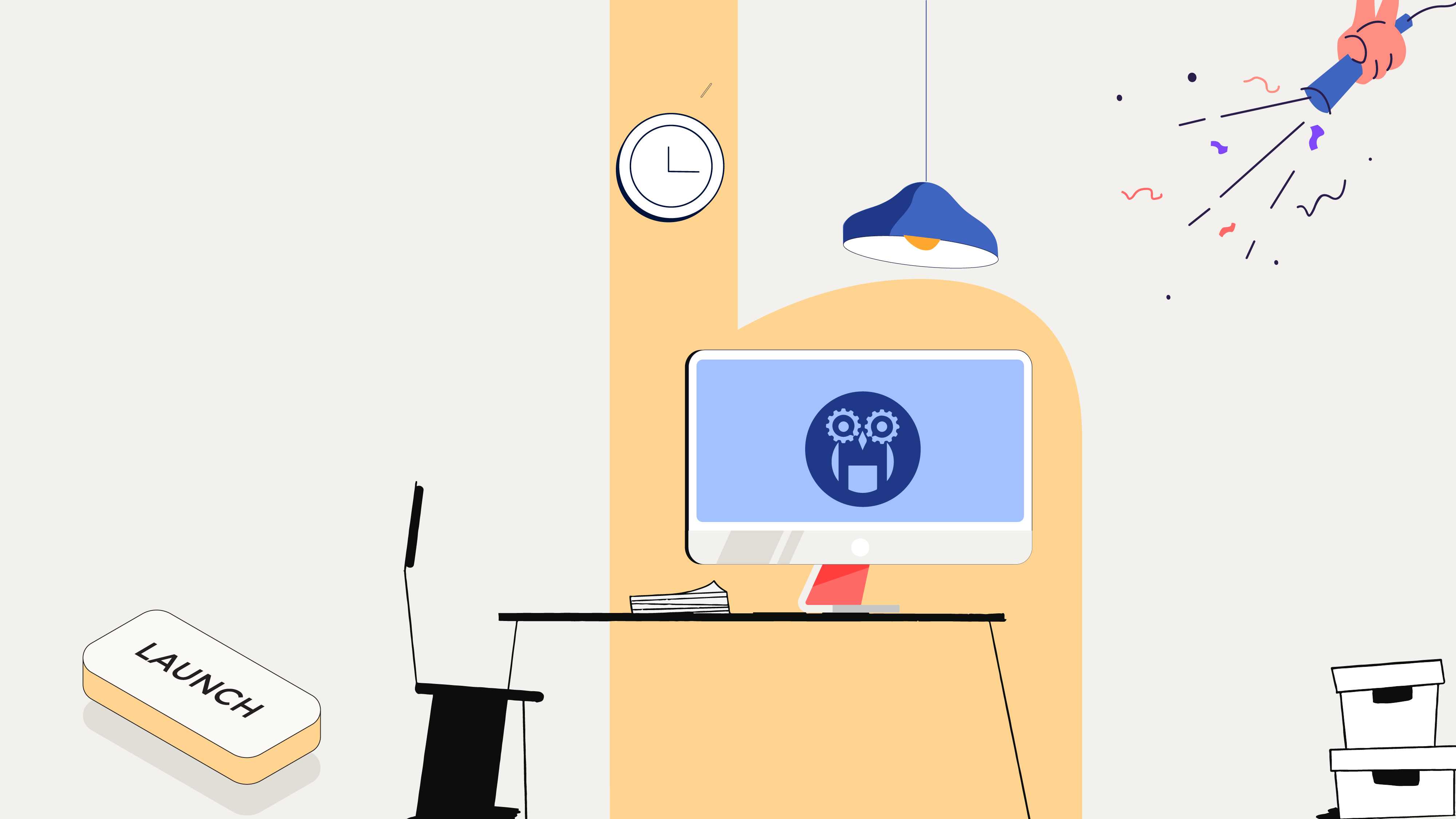
Introducing Hasper: LLM-powered Engine For Advanced Analytics
Over the last year, we have evolved from an MLops platform company that gave enterprises the ability to build and deploy machine learning for analytics teams, to an applied AI data products platform. Throughout this journey, our mission has remained consistent: to help organizations make better decisions using data. We’ve reached a pivotal moment in […]
Read More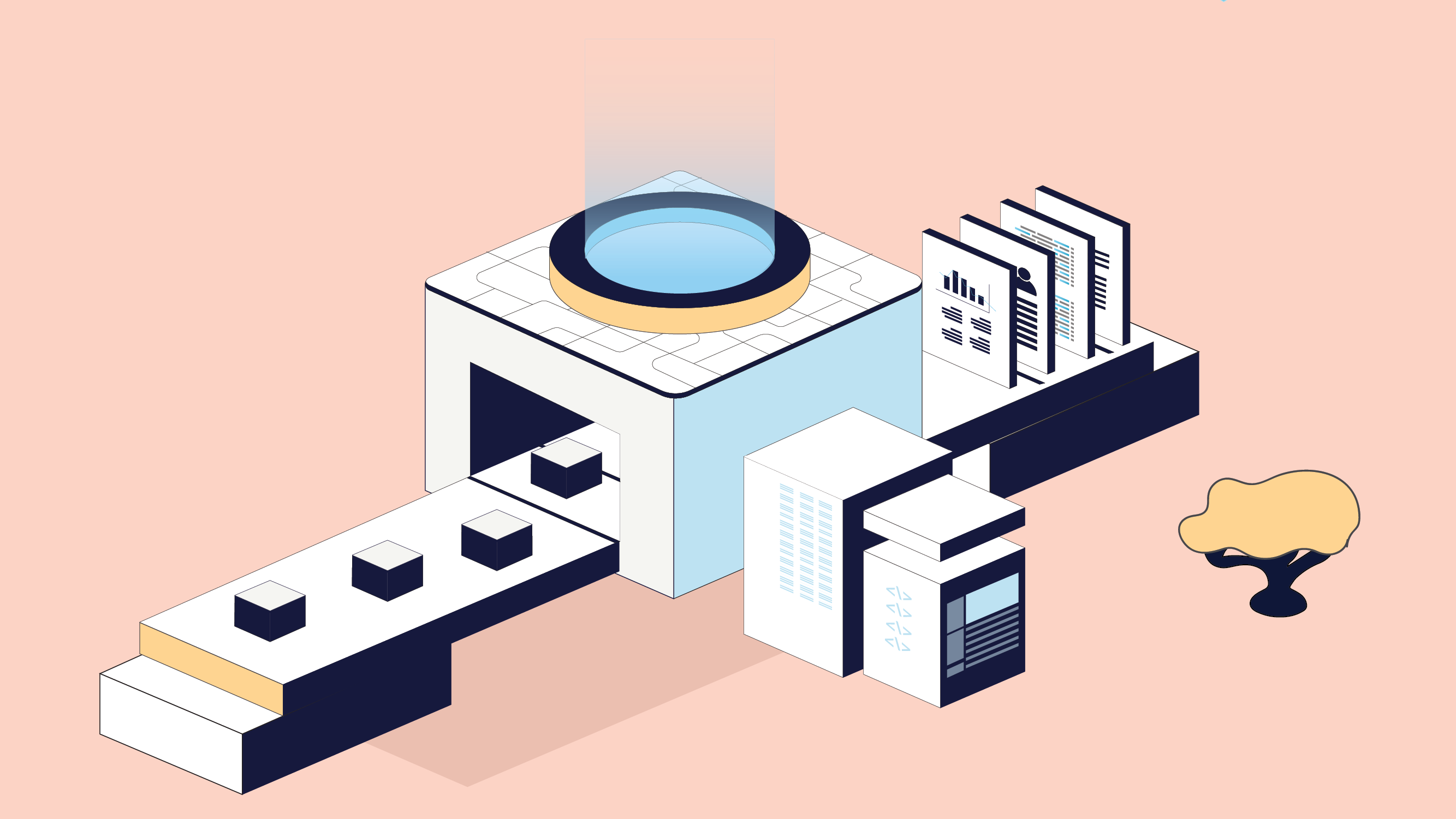
From Data to Decisions: How Generative AI is Transforming Enterprise Analytics
It’s the 24th century aboard the Starship Enterprise. Captain Jean-Luc Picard, in need of a break from the rigors of interstellar diplomacy, steps into the Holodeck. This isn’t just any room; it’s a technological marvel, a space where any scenario can be simulated, any world, any reality can come to life. Picard chooses a 1940s […]
Read More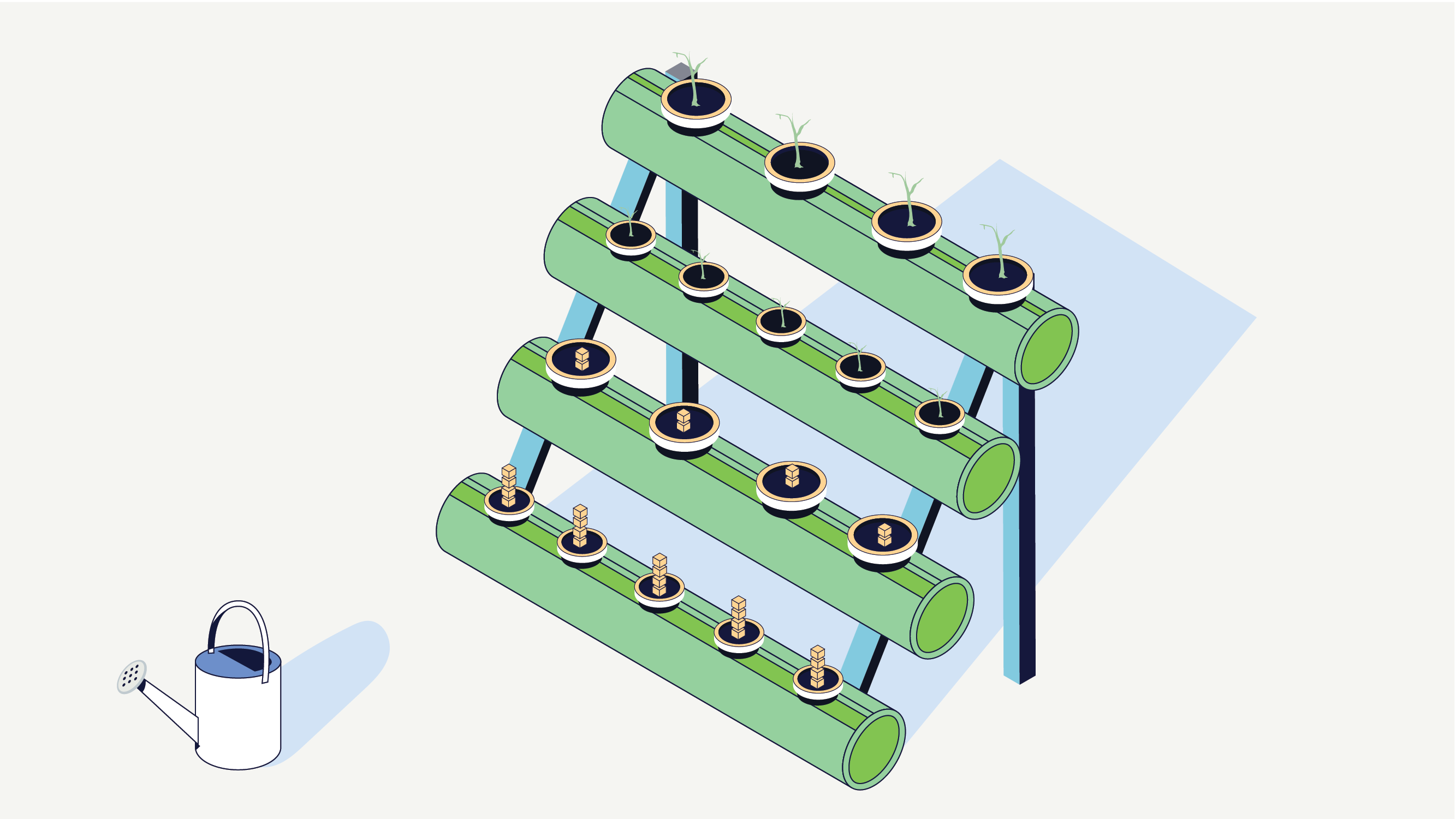
Generative AI in Insurance: Use Cases and Future Impact
What if the devastating Hurricane Katrina or Cyclone Nargis had been anticipated with greater precision, its impact mitigated by proactive insurance protocols? How would the landscape of life and health insurance change if underwriters could accurately simulate and understand the long-term health trends of populations? And what if reinsurers could preemptively navigate market collapses or […]
Read MoreStay updated on the latest and greatest at Scribble Data
Sign up to our newsletter and get exclusive access to our launches and updates