Imagine a world where a confluence of intelligent systems anticipate and cater to every want and need, seamlessly enhancing your day-to-day existence. A world where machine learning trickles into every cog that makes our world work, making it as essential and widespread as electricity.
There is a lot of optimism about machine learning (ML) in our current zeitgeist. Many people have proposed that machine learning will be everywhere. However, we would like to examine this proposition in depth.
How will it come to be that machine learning finds its way into every nook and corner of every single company? What are the challenges that we might face as we go down this path? If a world powered by ML is inevitable (we believe it is) then what are the real-world conditions that would support its ubiquity?
These are the questions that we will attempt to answer in this piece.
What makes technology ubiquitous?
If you look back at human history, any technology that becomes ubiquitous such as cars or electricity has had a few unique characteristics.
- Their cost has reduced to the point of common affordability
- The technology has been relatively safe
- Governing bodies have emerged to develop and enforce standards and best practices for all players in the ecosystem of that technology
- There are economic factors and business models that make the technology viable, allowing different entities like power distribution companies and appliance manufacturers to sustain and thrive within the ecosystem.
These attributes are common for every technology that has become an indispensable part of our lives such as the Internet, computer chips, transportation and so on. They are called general-purpose technologies because they touch every part of the economy.
Machine Learning Becoming Ubiquitous Is Inevitable
Machine learning appeals to our innate need for efficiency and problem-solving, which is a fundamental component of human nature, and lends credibility to the notion that it will eventually become widely used. According to numerous research studies, we have a natural propensity to look for patterns and connections, which is at the heart of machine learning algorithms.
Let’s just consider the last 400 years of human technological innovation. The technologies our species has developed such as pens, guns and automobiles have sprung forth to function as extensions of our capabilities. They are created to multiply the capabilities of individuals.
For example,
We want to travel from place A to B, but we can’t walk or run fast enough. So, we built cars.
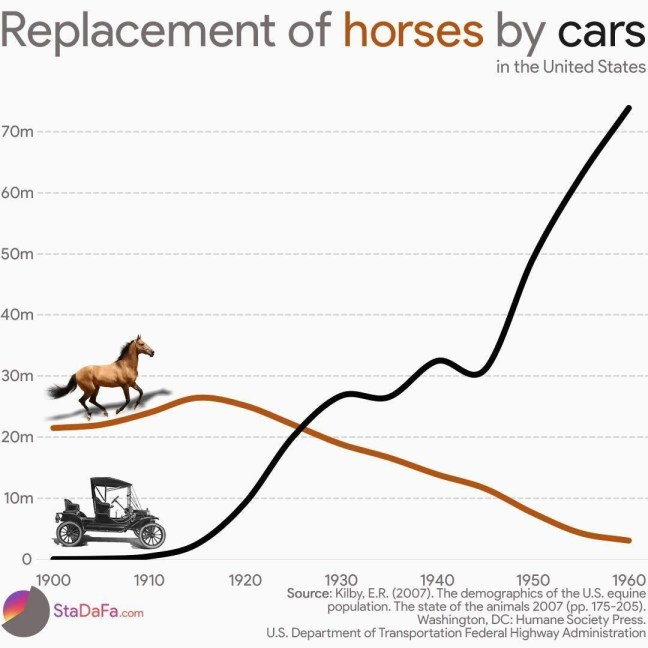
Source: Green Project Management, Omnirobotic
We manually operated our fans but at a point they simply weren’t efficient enough. So, we built electricity-powered fans.
These technologies function as a sort of exoskeleton, enabling us to transcend our limits and give us superpowers like the ability to traverse massive distances and lift incredibly heavy things. There’s one major frontier left – our MIND.
Unlocking The Final Frontier
We’re still in the early days of building an augmentation for our mind, but our technological focus is moving towards accelerating this process.
As humans, the complex world we live in requires us to think deeper, longer and faster. However, our mental capabilities have an upper bound – we can only remember a limited amount and have a limited number of things we can focus on. We also tend to have a lot of biases.
A system which becomes our mental augmentation in the new world will have to compensate for our biases and give us new tools to work with.
The world today is gargantuan in its complexity. The rise of global interconnectivity and interdependence through an ever-growing array of physical and virtual networks and the number of people, ideas, goods and information that pass through these networks is unprecedented. With an increase in complexity, we’re also seeing a rise in competition. It is harder for organizations to remain successful and top the charts as industry leaders in today’s environment. Impending risks like global warming, ecosystem collapses and modern problems like disinformation/misinformation are very prevalent. We need to rise up to the challenge.
Across the board – Engineers, painters, academics, and every other activity that covers white-collar jobs or creative pursuits can benefit from individual skill development. A painter can use an AI assistant to paint better. Coders can use AI tools to write better code.
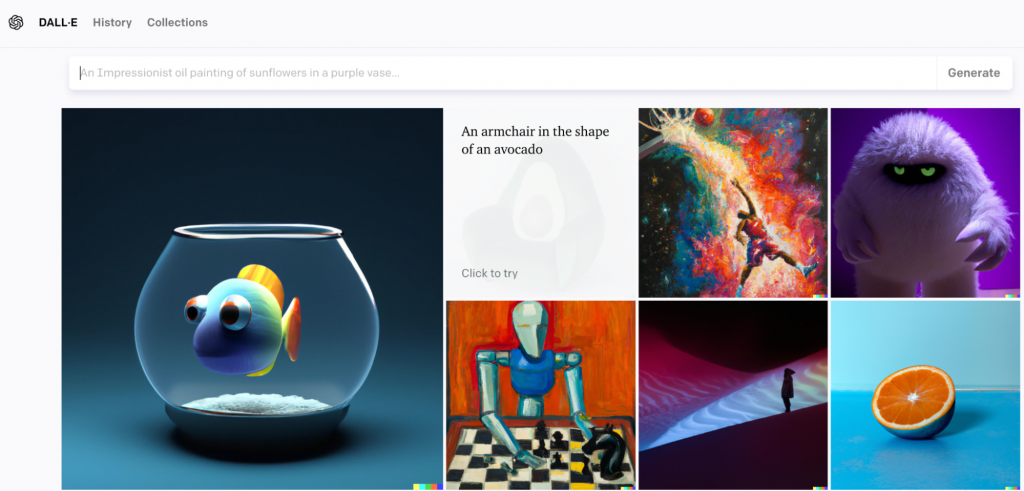
DALL-E, OpenAI’s Deep learning models that can generate digital images from natural language descriptions, called “prompts”, Source: OpenAI
This is where the need for ubiquity of ML makes itself very apparent. As decision-making machines, human beings are already always thinking about how to make better decisions, and ML will radically accelerate our quest to solve problems and step into a better future.
We Don’t Know What We Don’t Know
At Scribble Data, since our work has spanned so many diverse sectors, we have observed a pattern that has become very clear over the years – our expertise is shallower than we think and machines can help more than we might expect.
What do we mean by shallow expertise? Well, the siloed nature of a lot of current-day organizations creates redundancies and tunnel vision. As a result, staff understanding of organizational performance, their product and customers is less than expected.
We also discovered that our professions are rule-based to a fault. Once we form an opinion (Apple A is better than Apple B), we resist change in the presence of new information. We overestimate our knowledge, the accuracy of said knowledge and the ability to recall and update all this knowledge.
Healthcare is a classic example to demonstrate this phenomenon. Most of the diagnostic rule systems that have been developed in the healthcare industry have been refuted and updated several times over as our understanding of the human body has grown. Moreover, most medical recommendations are more prescriptive and rule-based than required, and do not evolve to reflect new understanding of our bodies, the mind-body connection, and human-ecosystem linkages.
Why ML Is Uniquely Suited to Be the Solution
Let’s try to imagine in widget form what the answer would be to all of the problems we’ve outlined so far. It would have to be a statistical box that will look at current and historical data and extract knowledge out of it. Today, we call this process “ML”. Tomorrow, we could call it “causal learning” or “deep learning”. We need not get bogged down in the semantics.
The solution will be able to squeeze insights out of data, and since we’re all collecting boatloads of data from the technology that has been deployed everywhere it will have a lot of data to process. So, ML is here to stay.
The Economics of Ubiquitous Machine Learning
Now that we’ve presented a theoretical case for ubiquity, let us think about a challenge that we must solve collectively. We have to think about what real-world economic conditions would have to be in place to foster global acceptance of ML.
The Technological Diffusion Cycle
If the past is prologue, technological adoption usually follows a specific cycle.
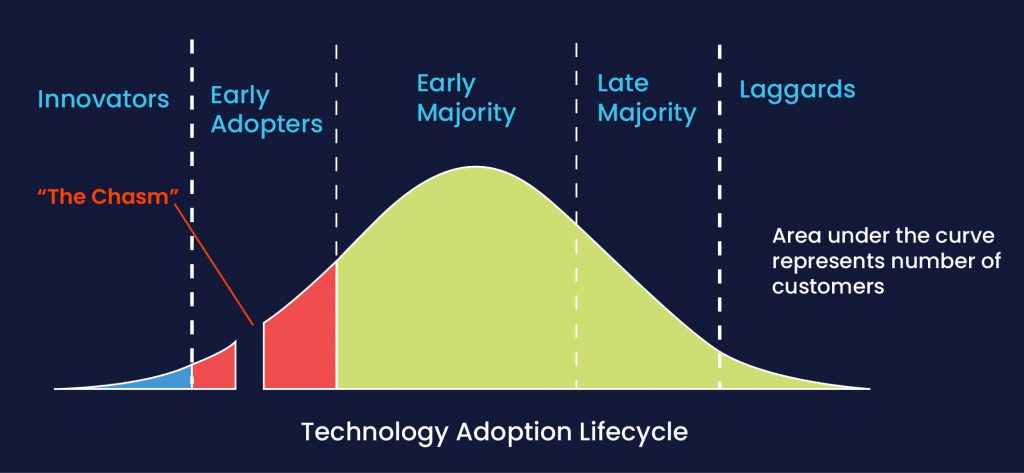
Technology Adoption Lifecycle, ” The Chasm “, (Moore, 1991)
In the early days of a technological innovation, the typical consumers are edge-cases. They are either rich people, powerful organizations such as the military or influential people such as sportsmen. They have very specific use cases, and they are willing to pay the most for advances in technology.
After establishing the value for these outliers, we get simpler versions of the technology. Before the Ford Model T took the consumer world by storm, there were other car companies. They were simply catering to a different group of people – the 1%.
Eventually, we went from building exclusive cars for rich people in the early 1900s to making it ubiquitous through the Model T and subsequent products. This industrial scale manufacturing to serve the masses, the 99%, was only made possible due to a number of innovations such as unique pricing models, assembly lines, training of workers in factories and loan mechanisms.
Where we are in the ML space is the equivalent of the automobile industry at the turn of the 1900s. There have been indications and glimpses of a Model-T equivalent. What we do know is that we want something different than what we’ve done so far.
The mental model that we currently have essentially came out of Uber – large engineering teams, a complex setup, real-time data etc. What we know now is that there are other types of systems we can build. The simple reason being that the economics does not scale. After all these years, we only have a few Uber-like companies due to the sheer complexity of keeping such a system functioning as it was intended to.
Let’s consider Uber’s incredible Michelangelo platform, which was instrumental in enabling Uber to scale up to 1000s of production models in just a few years. However, the restrictive cost and the amount of tech talent required to build an ML platform or feature store made it accessible only to a select few tech giants like Uber, Google, and Amazon. A number of companies spun off from these tech giants. Mainly because there was a need to democratize getting models to production and to make feature stores accessible to every organization.
However, the complexity of making things like a power plant will continue to create a natural barrier to entry into the ML world. There will be a group of people whose job it is to make the power plant and maintain it while the rest of us can focus on other problems. Some companies, like OpenAI, will function as de facto power plants of the ML world.
How Will ML Technology Get Cheaper?
Ubiquity implies that ML will be available to everyone – moms, children, office goers and across every strata of society. For it to be widely available, it needs to be cheaper.
There are several costs involved in machine learning – cost of data collection, cost of people, cost of computation and the cost of the skilled staff. The only way to make ML cheaper is to somehow reduce the cost of the individual.
As we see it, there are several different ways to amortize the cost of ML.
-
Generalized Shared Model
One way of reducing the cost is to have the Googles and Open AIs of the world – massive, complex technological products with 1000s of servers, high-paid engineers and so forth. A handful of these organizations will have the economic capabilities to build expensive tech that can service millions of users.
-
Domain-Specific Shared Model
Another way of distributing the cost is to have a shared ML model within organizations that build similar types of products or offer similar services. For example, if there is a third-party which develops an ML model that can predict and nip fraudulent Fintech transactions at the right time, it can be licensed by several companies within the space.
-
Build ML Into the Product
If you are an expense management company, you can have a data science team which will embed machine learning into the product such that whenever you submit an expense, it will be automatically categorized for you.
-
Full Stack at High-End – Centralized ML Platform
Solutions like Uber’s Michelangelo could be built to amortize the cost of development, deployment, and operations of the high end models that use complex algorithms and large volumes of data. These platforms reduce costs along multiple dimensions:
- Software development: All these ML solutions have pre-built software integrations and APIs reduce cost of development
- DevOps: Once the pieces of software have been developed, the platforms enables users to embed them into an application/API/Dockers easily without requiring high end ML engineers
- Lifecycle Management: A number of modules and templates are provided to reduce the cost at each step of the development and operations.
-
Full Stack on the Long Tail
The challenge with centralized ML platforms is that it is designed for scaling compute. The resulting designs tend to have high cost, limited flexibility, and high skill requirements.
If you take a typical company, the problems and challenges they face are very unique to that company, numerous, and low to medium complexity. Teams can build platforms that are optimized for scaling data products instead of data sizes.
Another important thing we are optimizing for is rapid consumption, making it very easy for end users to consume these products. We are also looking to reduce the friction towards consuming all of this data.
The Road to Ubiquity
Whichever shape the future of the ML-verse takes, we will be building systems with great economics because without it, we won’t get to ubiquity. We’ll need more than just Open AI to sufficiently revolutionize the world. As we’ve seen with healthcare, there are limits to the value you can derive from global public knowledge. Open AI can’t account for connection issues or problems that may be unique to your business and geolocation.
Another thing we think will happen is that ML acceptance will scale incredibly fast. It took approximately 40-50 years from the 1870s to around 1920 for the whole of the United States to be electrified. In developing nations like India, it took until the 1960s for the electrical revolution to take root.
We’re in the early days of the ML revolution. By my estimation, this started around the year 2010 which means we are in the 12th year of the movement. If we were to follow the electric movement timeline, this would put it somewhere around 2050 for ubiquity. But, I think we are due for an exponential take-off in the next 10 years and we will reach our target sometime in the next 25 years, by 2035.
Efficiency is King
Our initial experience in delivering use cases and learning from them has shown us that there is a lot of scope for organizations to become more efficient. As a result, what we are likely to see is a small number of companies that are incredibly lean and efficient which will outperform larger organizations which aren’t as nimble to adapt and pivot.
Massive organizational structures tend to include many redundant positions which create minimal value. These jobs will become unviable over time and may become obsolete. With such an upending of the existing organizational structure, there will undoubtedly be a lot of concern over the widespread adoption of ML. Over time though, the economics will speak for itself, and ubiquitous ML will gain widespread acceptance.
In the early days of electricity, there was a lot of concern about the repercussions of improper installation and faulty usage. However, the economics was compelling enough to justify the distribution of electricity. We expect ML adoptance to go the same way. One thing we have seen about these kinds of technologies is that once they are deployed, they change the way business is done.
If OpenAI has taught us one thing, it is that there is a different way of doing business. That in and of itself is going to open up a lot of use cases in the coming decade. At Scribble Data, these longtail use cases are what we are going to focus on in the time to come.