The year is 2023, and Sarah, a data analyst at a leading tech firm, no longer spends hours writing complex SQL queries or sifting through vast datasets. Instead, she simply asks her data product, powered by a Large Language Model (LLM), “What were the sales trends last quarter?” and receives a comprehensive, human-like response. This isn’t a scene from a sci-fi movie; it’s the reality of how data products are evolving.
The way we interact with data is undergoing a seismic shift. Gone are the days when data was a static entity, locked away in spreadsheets and databases. Today, data is dynamic, interactive, and, thanks to advancements in AI/ML, increasingly conversational. This transformation is not just about making data more accessible; it’s about making it more human.
But how did we get here? And what does this mean for businesses, developers, and end-users? Join us as we explore the current trends in data product development, from the rise of real-time analytics to the game-changing impact of generative AI and LLMs, and discover what the future holds for this rapidly evolving field.
Big Data Analytics Trends: A New Dawn in Data Interaction
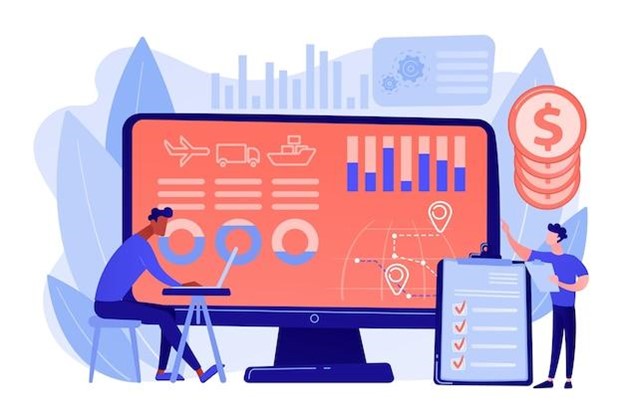
Ref: Freepik
As businesses generate and consume vast amounts of data daily, the tools and techniques to analyze this data have undergone significant transformations. Let’s delve into the key trends that are shaping the big data analytics landscape.
Data Lakes, Lakehouses, and Warehouses: The Evolution of Storage Solutions Data storage has undergone a significant transformation over the years. Initially, businesses relied on data warehouses, structured repositories designed for fast query performance. However, with the explosion of unstructured data from sources like social media and IoT devices, data lakes emerged as a solution. These lakes store vast amounts of raw data in its native format. The rise of data lakehouses, which combine the best of both worlds, is indicative of the industry’s move towards cloud-native solutions. These hybrid solutions offer both vast storage capabilities and structured querying benefits, aligning with the industry’s emphasis on cloud-native databases and advanced analytics.
Real-time Analytics and Stream Processing: The Need for Speed In the age of instant gratification, waiting for insights is no longer an option. Traditional batch processing is becoming obsolete in favor of stream processing, which analyzes data on-the-fly, as it’s generated. This shift is vital for applications like fraud detection, where immediate action is required. Tools like Apache Kafka and Apache Flink are at the forefront of this change. The importance of real-time analytics is further underscored by the increasing need for businesses to make decisions in the moment, leveraging continuous intelligence to act on insights as they emerge.
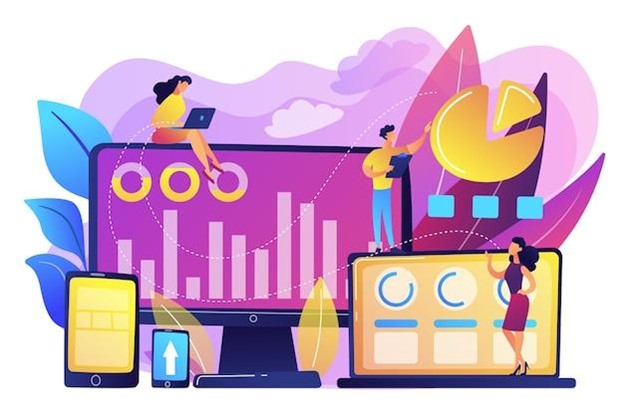
Ref: Freepik
Augmented Analytics: The Future of Data Interpretation Data interpretation is no longer confined to the realm of data scientists. Augmented analytics is democratizing data analysis by leveraging AI to automate data preparation and insight discovery. This trend is revolutionizing how businesses approach data, making insights more accessible and decision-making more informed. The integration of AI and machine learning in analytics platforms is enhancing the user experience, allowing for natural language queries and automated insights. Search volume for “AI analytics” has grown by 222% in the past five years, emphasizing the growing interest and adoption of this trend.
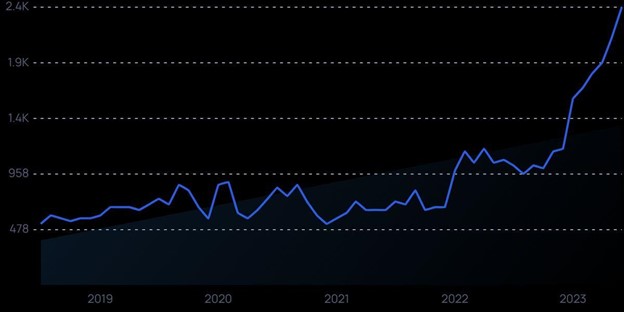
Ref: Freepik
More Use Cases For Edge Computing: Processing at the Source The digital world is generating data at an unprecedented rate. However, transmitting this data to centralized servers for processing can be slow and inefficient. Edge computing processes data right at its source, reducing latency and ensuring faster response times. By 2025, Gartner predicts over 50% of critical data will be processed outside of traditional data centers and clouds, highlighting the increasing significance of edge computing. As more devices get connected, the importance of edge computing will only grow, with businesses leveraging it for real-time analytics and decision-making.
For example, in a smart factory setup, Edge AI might be used to monitor equipment health in real-time. Sensors on the machinery would collect data, and local ML models would analyze this data to predict potential failures or maintenance needs. By processing data at the edge, the factory can take immediate corrective actions, minimizing downtime and maximizing efficiency.
Increasing Reliance On Data-As-A-Service (DaaS): Streamlined Data Management In today’s data-driven world, businesses need constant access to data. However, managing and maintaining in-house data infrastructure can be costly and complex. DaaS providers offer data collection, storage, and analysis services on a subscription basis, allowing businesses to access up-to-date data without the overheads of infrastructure management. The shift towards DaaS is set to accelerate, offering businesses a more flexible and cost-effective way to harness the power of data. The DaaS market is expected to grow at a CAGR of nearly 40% through 2027, adding $56.85 billion in value, indicating the rising reliance on this service.
The Democratization Of Data Systems: Empowering the Masses Data democratization is the idea that everyone, irrespective of their technical expertise, should have access to data. It’s about breaking down silos and making data accessible to all employees. This trend is not just about ease of use; it’s about fostering a data-driven culture. When data is at everyone’s fingertips, it leads to more informed decision-making across the board, driving innovation and growth. A Harvard Business Review survey found 97% of business leaders think democratizing data is crucial for business success, emphasizing the importance of this trend in the modern business landscape.
The Transformative Role of LLMs and Generative AI in Data Products
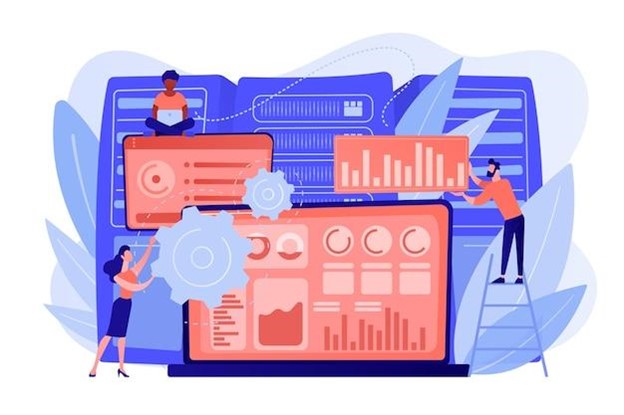
Ref: Freepik
Data Conversations with LLMs: In the traditional data analytics landscape, users had to rely on complex query languages or specialized tools to extract insights from databases. This often posed a challenge, especially for those without a technical background. However, the advent of LLMs is revolutionizing this space. LLMs facilitate a more interactive approach where data can “converse” with users. Instead of writing intricate queries, users can now simply ask questions and receive comprehensive answers. For instance, rather than querying a database about sales performance, one could ask, “How did our sales perform last quarter?” and receive a detailed narrative. Hasper, as an example, leverages this capability, allowing businesses to have natural conversations with their data, and making the process more intuitive and user-friendly.
Generative AI in Product Development: Generative AI is not just about creating art or music; it’s reshaping the way businesses approach data. These models can generate data that closely mirrors original datasets. This is invaluable for businesses looking to augment their datasets, simulate various scenarios, or even auto-generate reports. The real power of generative AI lies in its ability to provide enriched insights, bridge data gaps, and facilitate predictive analytics.
Productionization Challenges: While the capabilities of LLMs are groundbreaking, integrating them seamlessly into business operations is not without challenges. Ensuring that the generated insights are not just advanced but also relevant and actionable is crucial. This means customizing models for specific business needs, continuously training them and establishing robust feedback mechanisms. Hasper, recognizing these challenges, offers a platform that’s both technologically advanced and adaptable, ensuring businesses derive insights that are not just informative but also actionable.
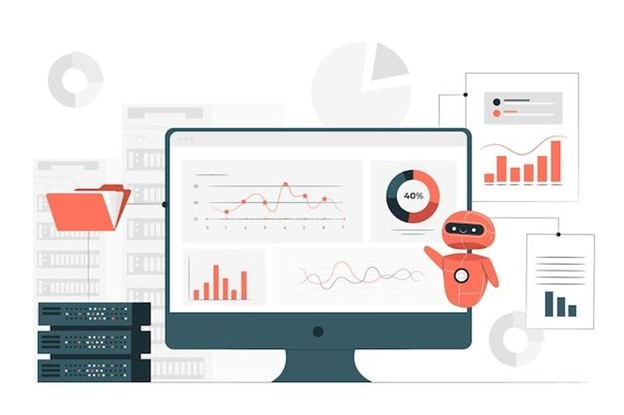
Ref: Freepik
Enhanced User Experience with LLMs: The transition to LLMs in data products represents a significant shift in user experience. Instead of static charts and tables, LLMs offer interactive and conversational interfaces. This evolution in data interaction makes the process more engaging and user-centric, allowing for a deeper exploration of data insights in a conversational manner.
Ethical Considerations: The integration of LLMs and generative AI into data products brings to the forefront several ethical considerations. It’s crucial to ensure the accuracy of generated content, avoid biases in data interpretation, and maintain transparency in how insights are derived. As these technologies become more prevalent, addressing these ethical concerns becomes paramount to maintaining trust and authenticity.
Data Product Development Trends
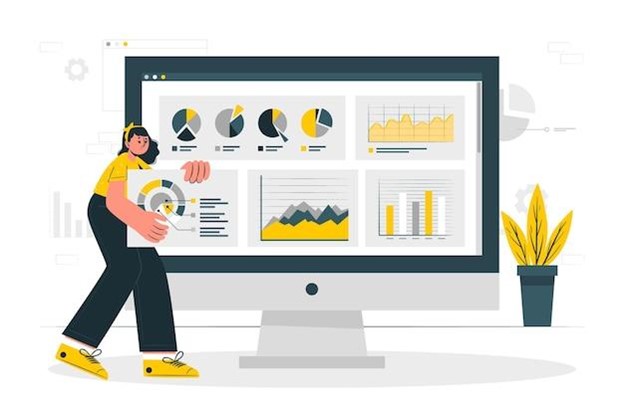
Ref: Freepik
Embedded Analytics: In the evolving landscape of data product development, the integration of analytics directly into applications has become a pivotal trend. Embedded analytics allows users to access real-time insights without switching between platforms or tools. This seamless integration enhances user experience by providing actionable insights right within the application they are using. For instance, a supply chain management application might embed analytics to instantly show inventory levels, demand forecasts, and potential bottlenecks, enabling managers to make informed decisions on-the-fly.
Interactive Dashboards: Visualization is a powerful tool in data interpretation. With the rise of big data, there’s a growing need for more intuitive ways to represent and explore complex datasets. Enter interactive dashboards. These advanced visualization tools go beyond static charts and graphs. They allow users to interact with data, drill down into specifics, and even run ad-hoc queries. For example, an interactive dashboard for a retail business might allow managers to click on a region to see sales data for individual stores, then further click on a store to see product-wise sales, all in a visually engaging manner.
DataOps: As data product development becomes more complex, there’s an increasing emphasis on streamlining operations and ensuring data quality. DataOps, inspired by the principles of DevOps, focuses on improving the entire data lifecycle. It emphasizes collaboration between data scientists, engineers, and business stakeholders. The goal is to ensure that data products are developed efficiently, with a focus on continuous integration, testing, and deployment. By adopting DataOps principles, organizations aim to reduce the time-to-market for their data products while ensuring that the insights generated are accurate and reliable. For instance, a financial institution might use DataOps to ensure that its credit scoring models are updated regularly with fresh data, tested rigorously, and deployed seamlessly to their loan approval applications.
Serverless Data Analytics: The cloud computing landscape is undergoing a significant transformation with the rise of serverless architectures. Unlike traditional cloud setups where resources are pre-allocated and billed continuously, serverless architectures allow developers to run code in response to events without managing the underlying infrastructure. This means that resources are allocated dynamically as needed and users are billed only for the actual compute time they consume. In the realm of data analytics, this translates to on-demand processing power, enabling businesses to run complex analytics tasks without maintaining dedicated servers. The benefits are manifold: reduced operational overhead, cost savings, and the ability to scale analytics workloads seamlessly. For instance, a retail business might use serverless data analytics to process sales data during peak shopping seasons, ensuring timely insights without incurring the cost of idle resources during off-peak times.
Conclusion
As we’ve journeyed through the evolving landscape of data product development, it’s evident that the future of data interaction is not just about numbers and charts; it’s about narratives, conversations, and human-like engagements. The transformative role of LLMs, generative AI, cloud computing, and edge analytics signifies a departure from traditional methods and ushers in a new era where data is not just consumed but conversed with.
While the potential of these technologies is vast, it’s essential to approach them with a balanced perspective, acknowledging the challenges they present while harnessing their transformative capabilities. As we look ahead, the fusion of human intuition with machine intelligence will be the cornerstone of the next wave of data products.
As we step into this new era of data analytics, it’s worth exploring platforms like Hasper that are at the forefront of this revolution, bridging the gap between today’s needs and tomorrow’s possibilities. With its emphasis on natural language interactions and AI-driven insights, Hasper stands as a testament to the transformative potential of these technologies.