Machine learning and data science today are in a unique position where access to capital is often not the biggest barrier to success. Companies globally are continuing to invest into artificial intelligence to the tune of $140 billion, either to develop AI-native products or solutions or as a way to solve business problems and improve efficiency. Yet Gartner estimates that only 53% of artificial intelligence (AI) projects make it from prototypes to production. This indicates widespread failures occur well before several ambitious and exciting AI use cases can be deployed commercially. So what is breaking down between testing out an idea and building an initial prototype and taking the project to production? Often, it is a lack of an outcome focused data strategy and contextual expertise that proves to be the stumbling block.
People are at the core of technology, both as creators and users, and not the other way around. In the excitement of leveraging the incredible powers of AI, companies are apt to make investments without putting in place prioritization frameworks or other guardrails to ensure the success of the project. This may be seen through money pumped into areas that are not well-suited for ML applications or projects losing their way with the actual development process due to poor strategy and tactics.
Context and expertise are the other areas that are not prioritized in executions that result in failure. A data science problem is first a business problem and that context must always stay in the foreground. Expertise in data science is thought of as technical expertise but non-technical members like process experts or subject matter experts add a different dimension to the entire MLOps workflow, which is the process of taking ML to production as well as managing and maintaining the models.
Data science teams are fragmented
Companies of all shapes and sizes have invested into building data science and AI teams. But there is not always a clear direction or vision of how these need to be structured for the most optimal performance across the organization, especially when these functions are layered on top of existing business units and structures.
Some companies adopt the hub and spoke approach where the AI and business analytics experts who work with different teams are grouped into one team, while others look to the centralized model where the AI team is one team in itself. In a completely decentralized model, the AI teams sit within the business unit they support.
While each of these has its advantages and disadvantages, it is important to ensure that the data science expertise is not siloed and at an arm’s length from the core operations of the company or business unit. An open exchange of ideas and feedback must exist for the AI teams to build the most impactful products and for the business-focused teams to leverage this technology in the most optimal way.
Lack of Business Context
A challenge that stems from these data science silos and is worsened by other factors is the lack of business context that informs AI operations. Machine learning models are worked on by people to be used by people, directly or indirectly. It is only logical then that ML should be used to crack real-world business problems and to power decision intelligence, and not treated as a purely mathematical challenge to be solved. Every row in a dataset represents actual people, entities or some real-life scenario and this context must be remembered at all times. Subject matter experts in the organization are also invaluable in providing more context to the problem statement. The interdisciplinary nature of AI is sometimes overlooked, but non-technical experts can have a significant contribution, particularly in areas that need some level of subject matter expertise.
For a team building a customer assistance model for e-commerce, for example, working with customer-facing retail teams on a regular basis is crucial. They will then understand the patterns and trends that the subject matter experts are seeing and can take that insight into the AI development process and build and maintain a much more targeted solution.
An array of roles and responsibilities come with building a full-fledged AI team – data scientists, ML engineers, data engineers, software engineers. But it is not enough to hire the best and brightest in technical talent who can produce brilliant results from an academic perspective. Technology for technology’s sake is of lesser value if it does not solve the business problems at hand. The technical roles required must be mapped keeping in mind the outcome, and how to best meet the business goals.
The common thread that runs through all these is the importance of spending time thinking about the why – i.e why data science or business analytics is being leveraged. Be it reducing manual effort, saving costs, or improving efficiency, this overarching goal will guide the entire process. This is particularly true when AI enters core industries like manufacturing, retail, or financial services.
How businesses can get it right
Setting an outcome-focused data strategy
Management by objectives (MBO) is an industry-standard framework for managers to work with their employees, where a goal is set and then the means to achieve this goal are laid out, in a mutual discussion.
If we apply the same principle to an AI project, a clear understanding of the outcome must be the first step. This first critical step impacts all later ones including the selection of datasets, processes, required tools and partners, and infrastructure planning. Being hyper-targeted in setting this outcome will sharpen the lifecycle and produce more relevant and intelligent results. Reversing this order could result in repeated trial and error through the MLOps pipeline which ultimately is less useful in a fast-moving business context.
Selecting relevant inputs based on outcomes
The oft-repeated maxim ‘garbage in, garbage out’ has become even more ubiquitous recently since the role of data and its quality in the AI training process has become a pivotal focus of the ecosystem. AI leaders like Andrew Ng have stressed the importance of “data-centric AI” which he describes as “the discipline of systematically engineering the data needed to build a successful AI system”.
The careful selection of the right dataset for the context and thereafter a systematic process of data labeling and preparation for the training process can have an exponential impact on the final performance of the model.
In a recent article about Adversarial Data Science, we shared an example of an eCommerce company that suffered heavy losses from unsold inventory due to inaccurate data: they ordered high volumes of inventory of products across their catalog because their analytics data showed visitors browsing through multiple products on its platform. However, the sales data showed that they weren’t selling enough products even when the site visits and engagement rate was high. They found that their numbers were wrong due to a mistake in their incoming data — the QA team left a crawler on the website, which was almost poisoning the understanding of demand. Because of this crawler, 1/3rd of their traffic was nonexistent, leading to inventory mismanagement and lost revenue.
Standardizing AI-business team collaboration processes
Business units and AI teams are most often working together to meet a common goal, for example reducing the time taken to complete the customer onboarding process. For this goal to be met, it is imperative to build an environment of trust and collaboration. The business team members must not view AI and its practitioners as a threat or as a delinked technology arm. In turn, the data science and AI teams must recognize that business units are their internal customers and, as such, their expertise and feedback is valuable. Furthermore, AI solutions are data-driven and, unlike deterministic algorithms and heuristics that business teams are used to, often are implemented as black box architectures. To drive adoption, AI teams must defer to using explainable models when possible.
In an extension of this creator-customer logic, codifying cross-team collaboration and quality monitoring in the form of service-level agreements (SLAs) and Standard Operating Procedures (SOPs) can remove any ambiguity and ensure seamless functioning, especially when projects begin to scale.
AI projects in a production environment are fluid and agile. So putting down MLOps processes and nomenclature on paper forces stakeholders to evaluate how cross-functional operations are carried out and, where possible, remove redundancies and contradictions.
Leveraging tools and technology
When the process of developing an outcome-focused data strategy is complete and the appropriate datasets are identified, it is time to select and work with technologies that will help transform this vision into concrete data projects and eventually into business success in some form.
No modern organization, however large, has the resources to build and maintain tools for every part of the circuitous AI development process and relies on trusted partners for specialized functions. Adopting a strategic approach, driven by data and insights, helps in being more precise in tool selection, to supplement the specific areas where internal technologies are not enough.
At Scribble Data, our focus is on feature engineering. We empower teams who have identified a particular outcome and the data needed to build for that outcome. Our clients are able to productionize their valuable data and extract features that help them solve real-world business problems.
In one example, a nation-wide premium mall chain in India’s Tier I and Tier II cities wanted to better understand their customer base for increased engagement and growth. On the business side, they realized that the cost of acquiring new customers, i.e. attracting more traffic to the mall would be higher than the cost of offering incentives to existing visitors. They were looking to optimize the footfall and eventually store revenue in less-frequented areas of the mall.
With this specific outcome in mind, the management deployed 50 sensors all across the premises to track movement and activity. But they did not have the required data platform, infrastructure, or team to host and compute the data.
With Scribble’s Enrich tool, the mall was able to run data analysis on GBs of data from millions of observations from 25 sensors. It provided the ability to track over 600K visitors and segment them based on behavior, and determine 150 visitor metrics including entry path into the mall, frequency of visits, and time spent at various locations.
This data and pattern analysis enabled better ad locations and targeting of offers. The overall shopper experience was built upon with seating options, better lighting, and offers to direct shopper attention to the less busy sections of the mall.
(Read the full case study here.)
The road ahead 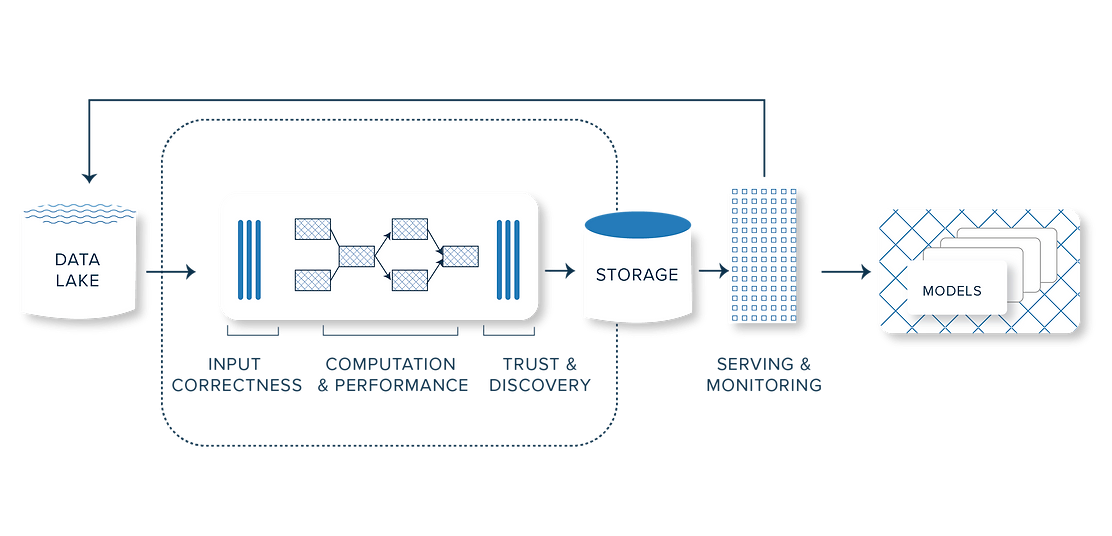
Scribble Data Enrich as a part of the MLOps stackScribble Enrich is used as a part of the broader MLOps technology stack. MLOps helps streamline the complexities of the ML lifecycle from ingestion all the way to production and validation. The model monitoring process, for example, can be made easier using Anodot, Flyte, or Seldon Core.
As the market moves to production and deployment and the emphasis on outcomes increases, the challenges also evolve. The challenges of getting an idea for an ML use case off the ground and those of scaling are very different. Working with the right partners for each of these operations gives you the best chance of productizing your AI project and not falling prey to the pitfalls that stall 47% of projects.
Keeping a continual focus on the business context and an outcome-focused data strategy ensures that an investment in AI has tangible and measurable results. At a macro level, it all goes back to the outcome that was set: has that been met? How much is the delta and how can the gap be bridged through data and model improvements? Analytics and dashboards offered by different tools also make it easier to track down the specific workflow that is causing problems.
But all this is made possible through the thorough planning and data strategy that must happen at the beginning of the digital transformation and AI journey. This might take a little longer than diving headlong into the AI world but this frontloading method can save time, effort, and money along the way. Using data analytics is an effective method but one that needs some level of investment. So it’s vitally important to take a calculated approach even early on to tap into the full potential of transformative technologies like AI and machine learning.