Resources
Blog posts
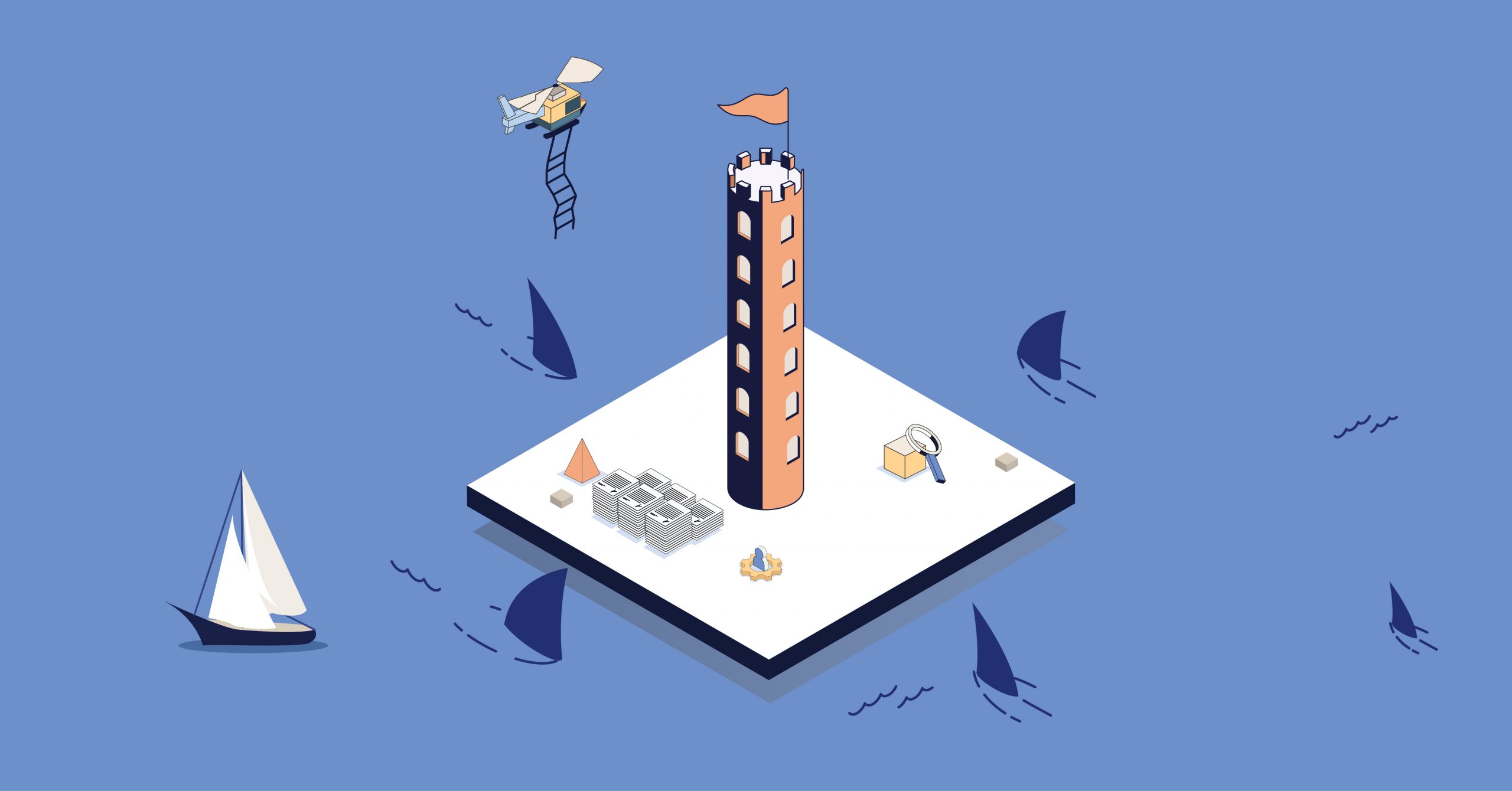
The Insurer’s Guide to Managing Risks in Employee Benefits
Every day, insurers in the employee benefits space walk a tightrope, balancing profitability against market demands, regulatory pressures, and evolving workforce dynamics. One misstep in risk assessment or management can trigger a domino effect, potentially wiping out years of hard-earned gains. In this high-stakes environment, risk isn’t just an abstract concept—it’s a tangible force that […]
Read More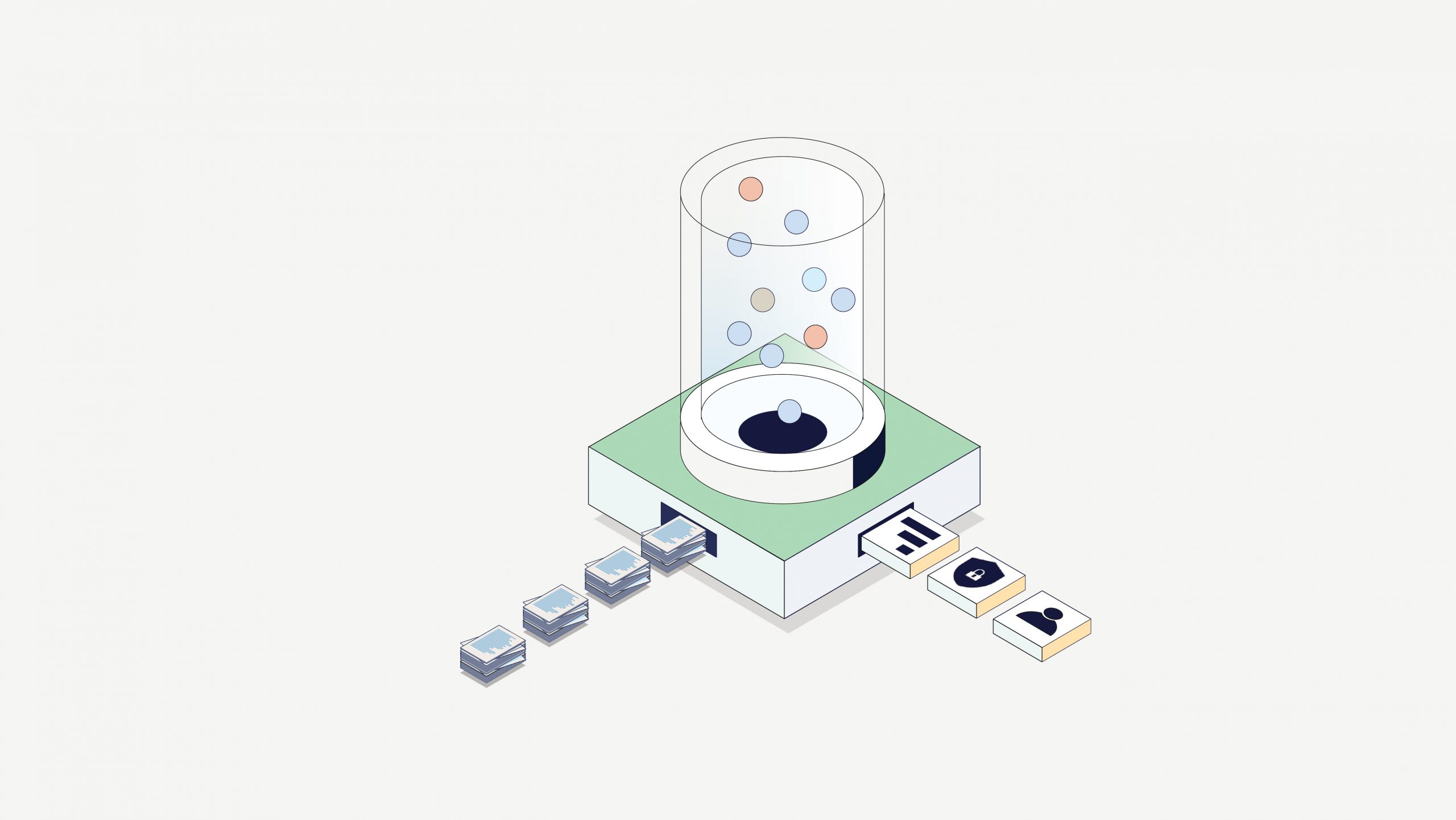
The Role of Technology in Employee Benefits Administration
Behind every benefits package is a human story. A new parent juggling childcare and career ambitions. A middle-aged employee managing a chronic condition while striving for peak performance. A near-retiree planning for the next chapter of life after decades of service. These aren’t just data points or policy numbers – they’re real people with complex […]
Read More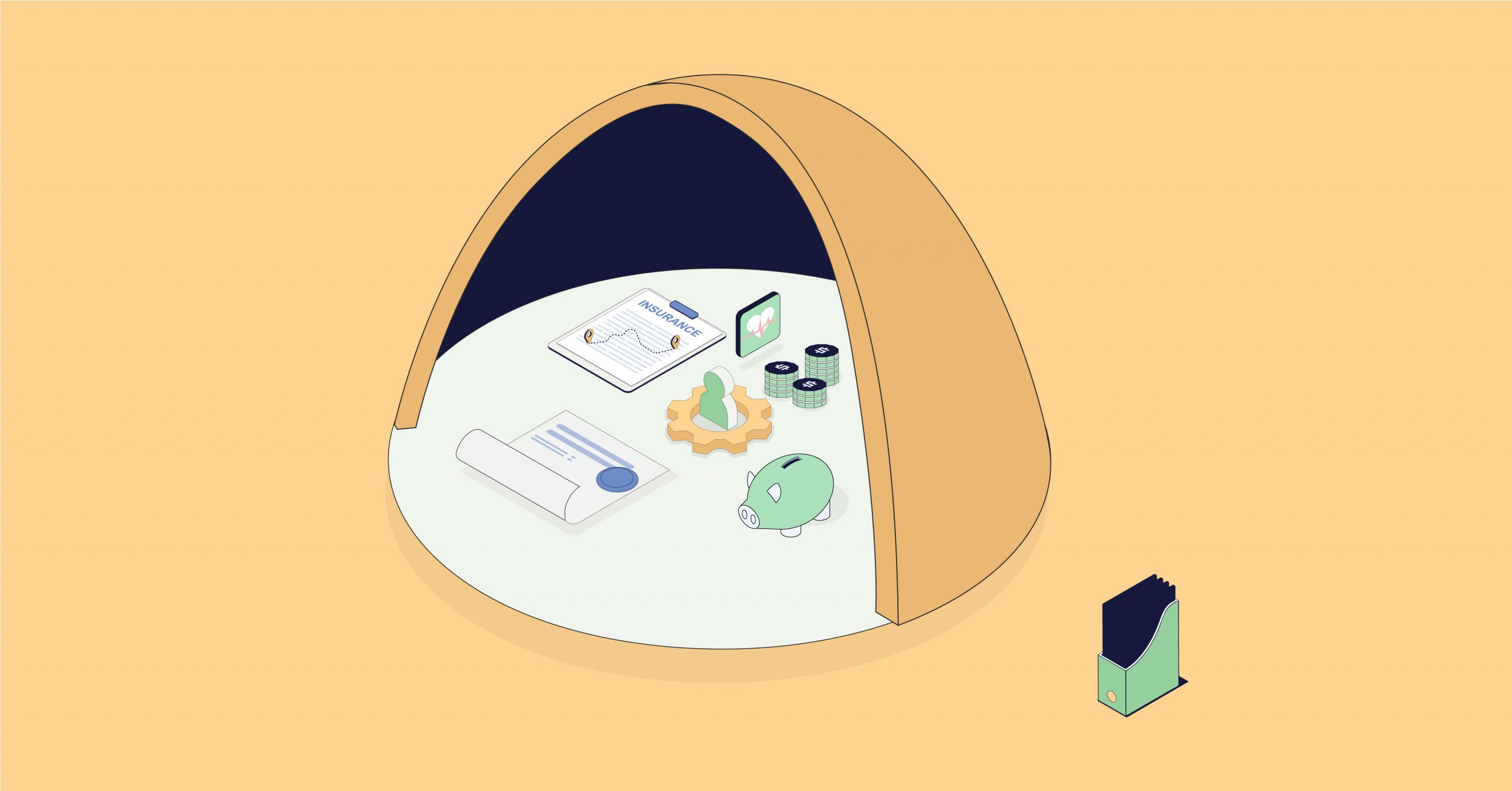
Understanding Employee Benefits in the UK: A Comprehensive Guide
In a nation where queuing is an art form and discussing the weather is a national pastime, the UK employee benefits landscape presents a uniquely British challenge. How does an employer stand out in a job market where statutory benefits are already more comprehensive than in many other countries? When everyone receives 28 days of […]
Read More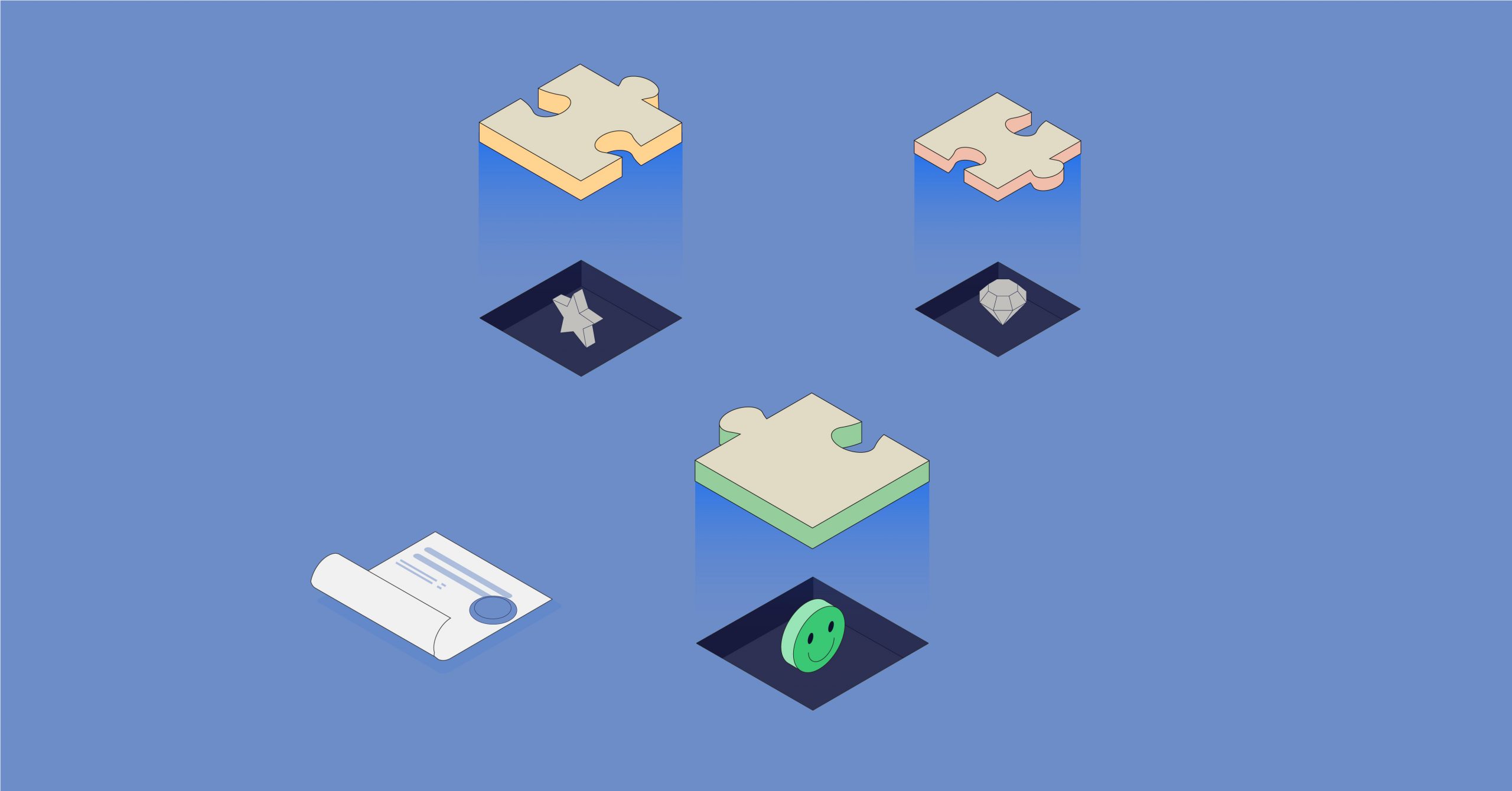
The U.S. Employee Benefits Landscape: A Complete Guide to Future Trends and Regulations
Let’s imagine a giant, glowing price tag hovering over a quintessential American neighborhood. On it, etched in bold letters, are the components of today’s American Dream: HOME OWNERSHIP: $374,900 HEALTHCARE: $22,221/year COLLEGE EDUCATION: $103,456 RETIREMENT: $1,120,000 WORK-LIFE BALANCE: PRICELESS Staggering, isn’t it? The cost of the American Dream has skyrocketed, leaving many wondering if it’s […]
Read More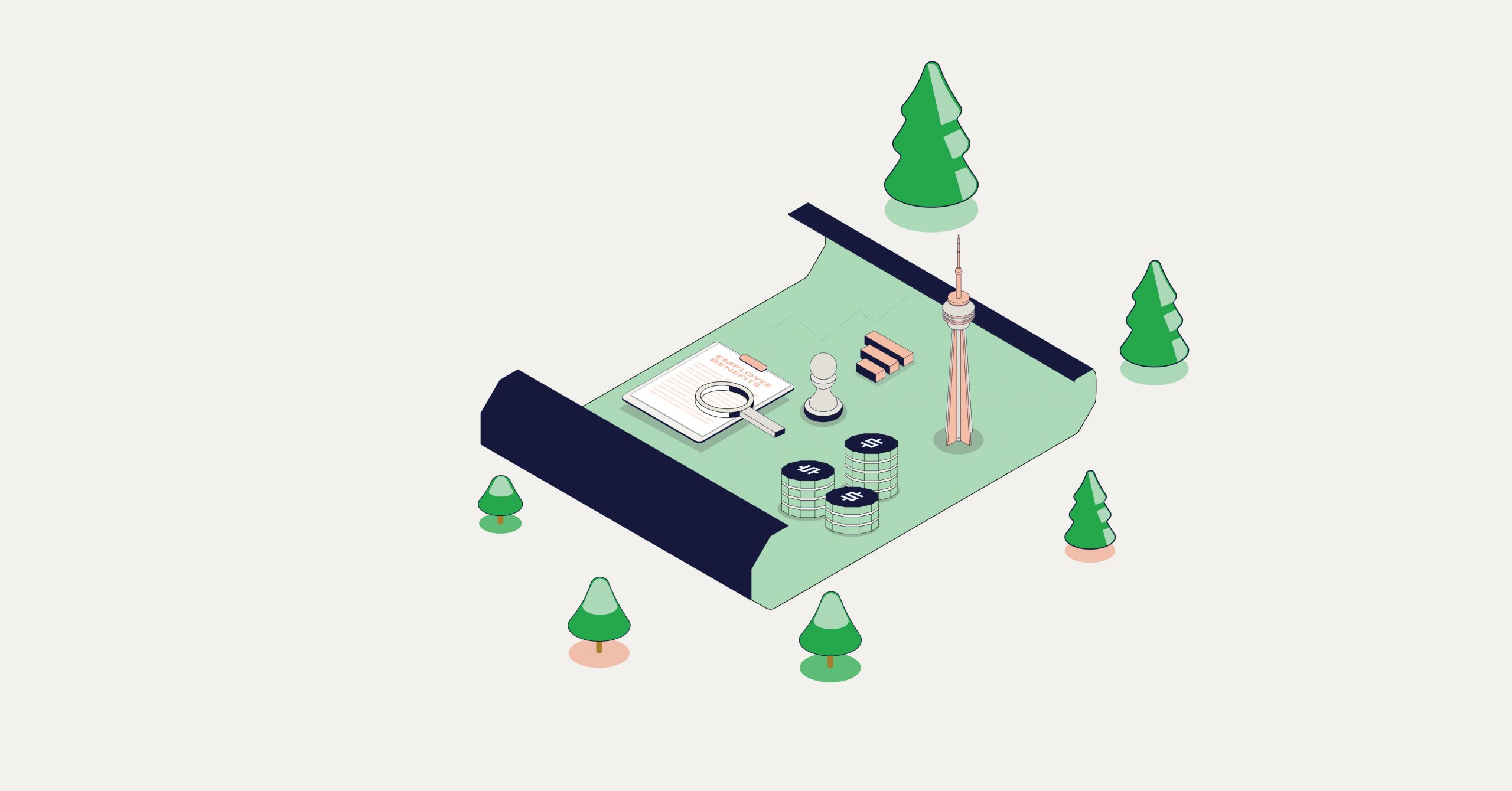
Future of Employee Benefits in Canada: Trends, Regulatory Landscape, and Opportunities
Sarah’s alarm chimes at 6:30 AM. She stretches, knowing a busy day lies ahead. But there’s no panic in her movements. No frantic mental math about doctor’s bills or retirement savings. Sarah, like millions of Canadians, starts her day wrapped in an invisible safety net. She checks her phone. A notification reminds her of a […]
Read More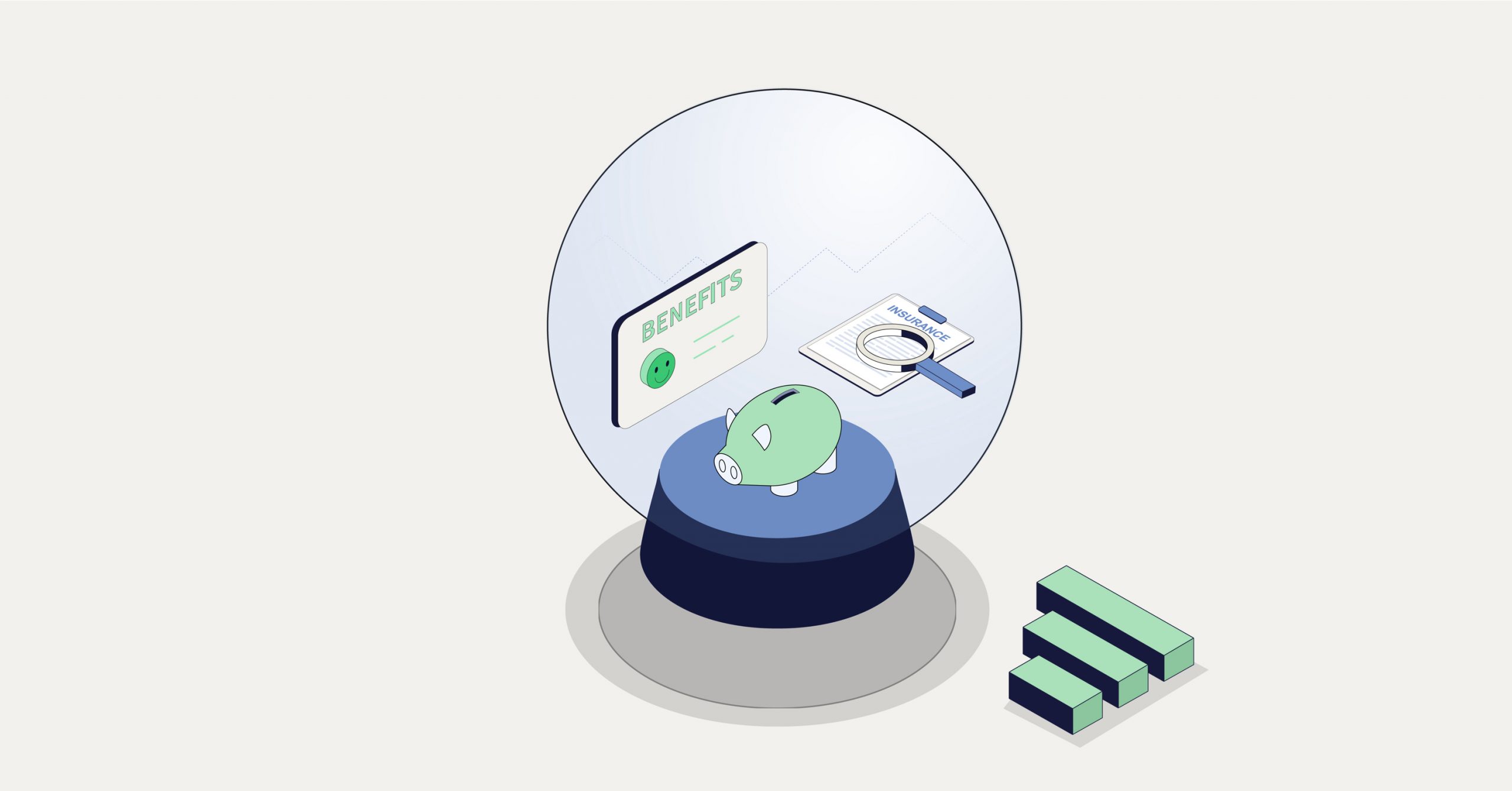
AI and the Future of Employee Benefits: A Complete Guide
The world of employee benefits is broken. Navigating the labyrinth of options, forms, and systems is a nightmare. Enrollment is a time-sink, and getting answers is a game of roulette with conflicting information at every turn. But in the age of AI, this does not have to be the reality. Forward-thinking enterprises and insurers are […]
Read More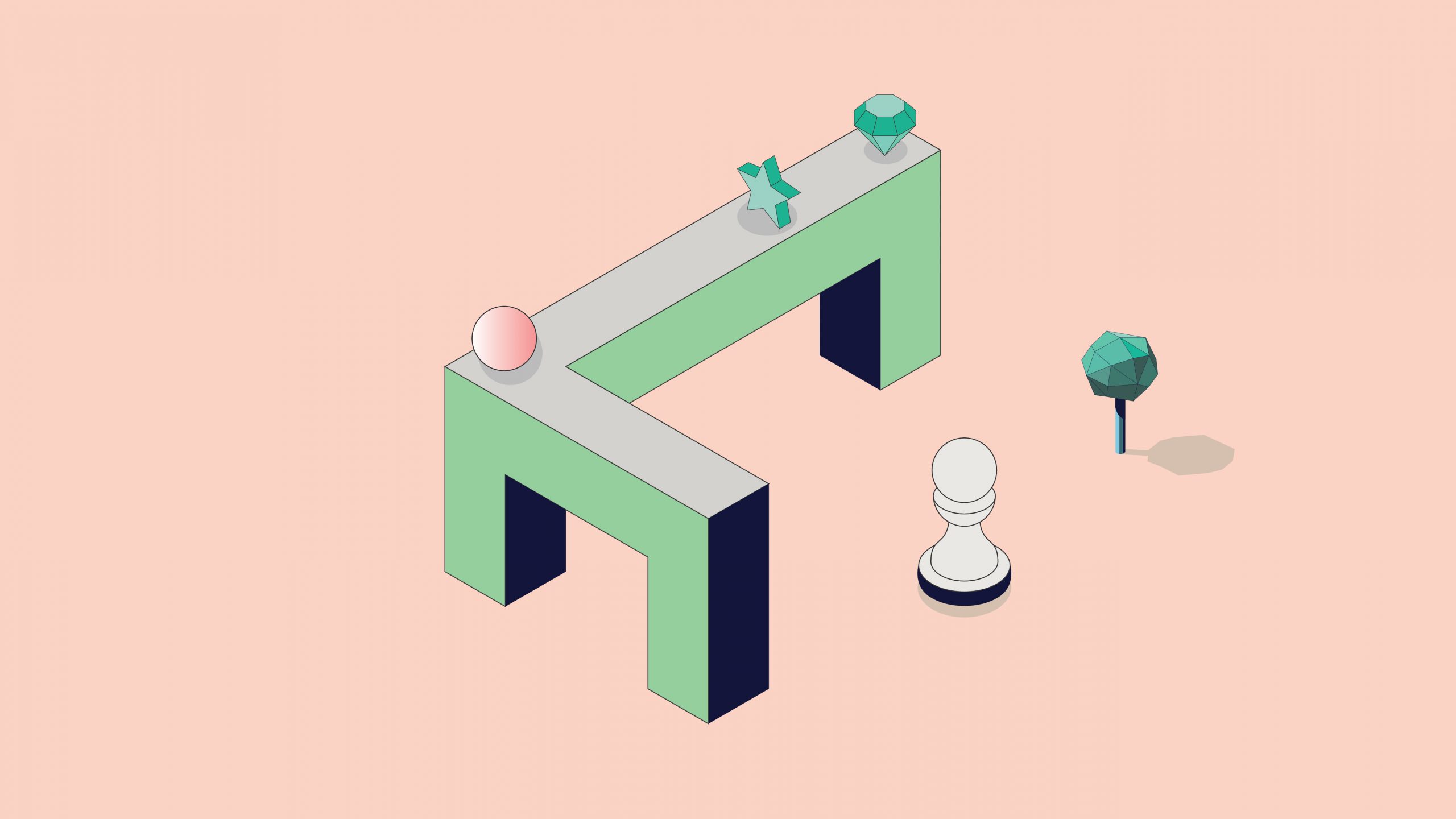
Employee Benefits Explained: History, Evolution, and Key Trends
Did you know that employee benefits can make up to 30% of an individual’s total compensation package? This staggering figure highlights the immense significance of benefits in the modern workplace. From attracting top talent to retaining loyal employees, benefits play a crucial role in the success of any organization. But the world of employee and […]
Read More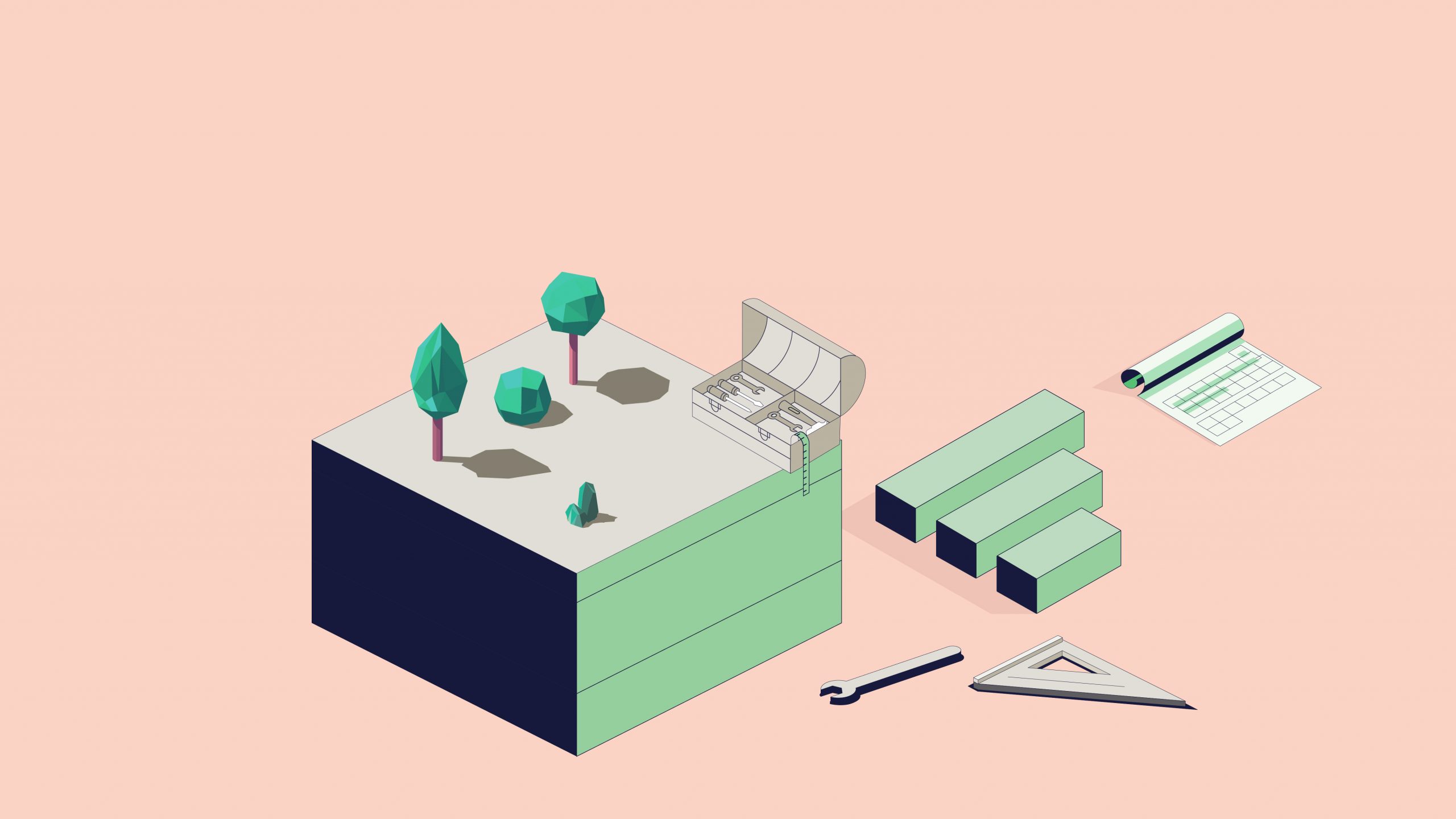
Top Asset Management Trends to Watch Out for in 2024
The year is 2024, and the world of asset management is in flux. A decade ago, few could have predicted the seismic shifts that would reshape the industry – from the rise of passive investing and the democratization of financial data to the existential threat of climate change and the disruptive power of artificial intelligence. […]
Read More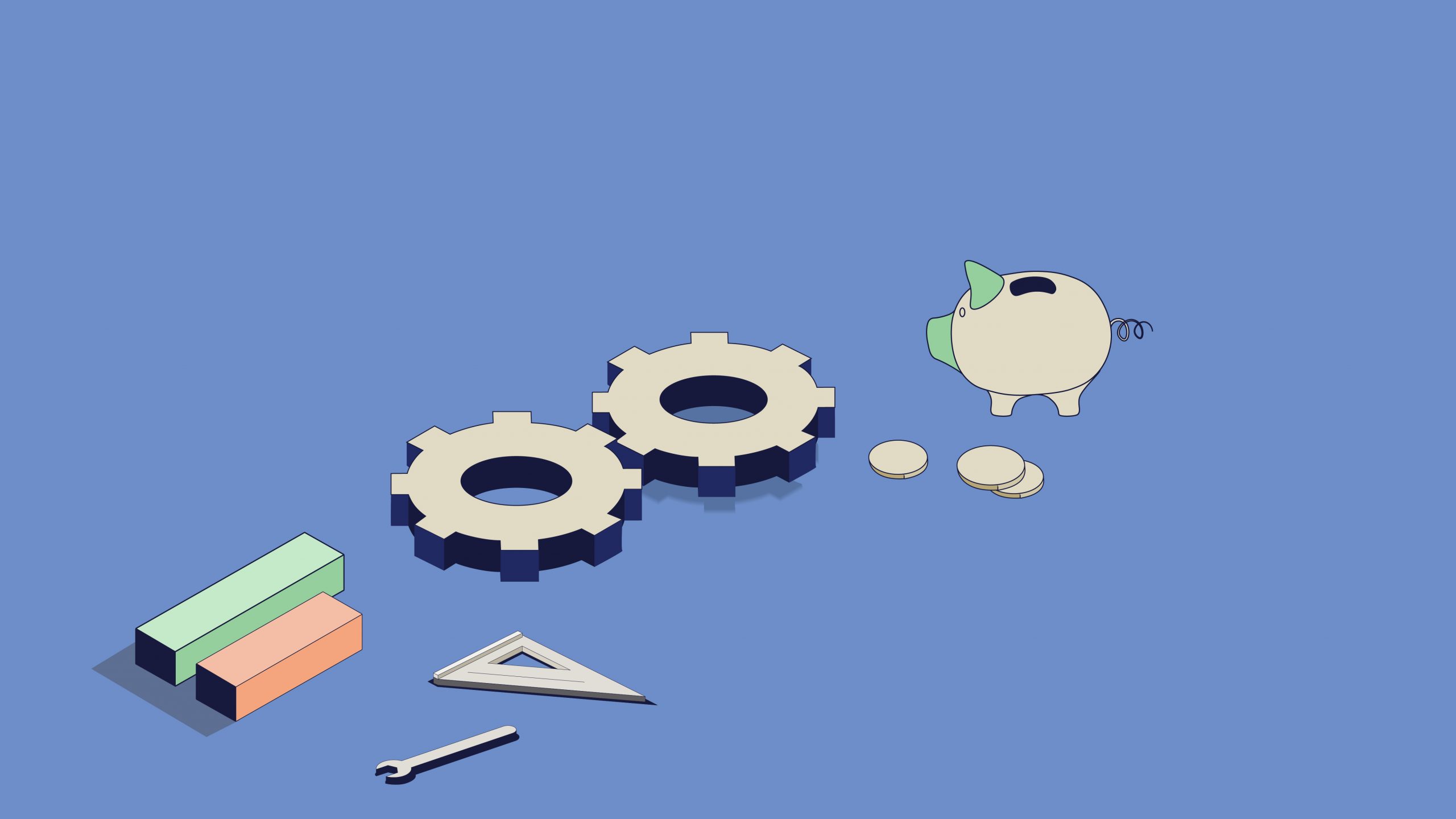
The Strategic Role of Longevity Swaps in Pension Fund Management
Octogenarians are becoming the norm. Centenarians are no longer a rarity. As the world’s population ages, the financial burden on pension plans and insurers grows. Traditional risk models are crumbling under the weight of this demographic shift. The solution? A groundbreaking financial instrument called the longevity swap. Longevity risk, the risk that people live longer […]
Read MoreCase Studies
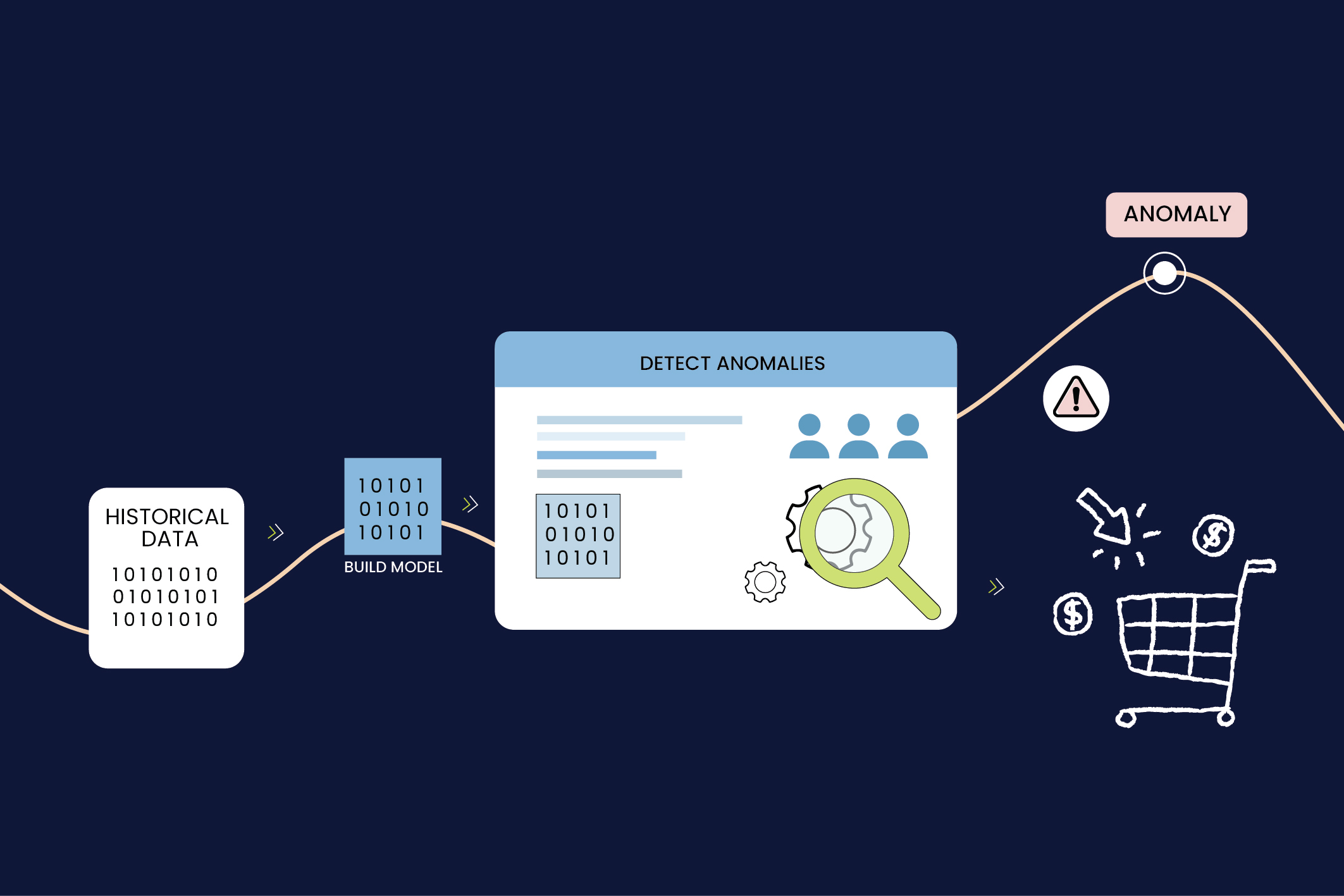
Finding Conversion Anomalies at a Large E-Commerce Firm
Learn how a leading multi-billion dollar e-commerce company used Enrich to identify anomalies in their conversion rates and to find out their causal factors.
Read More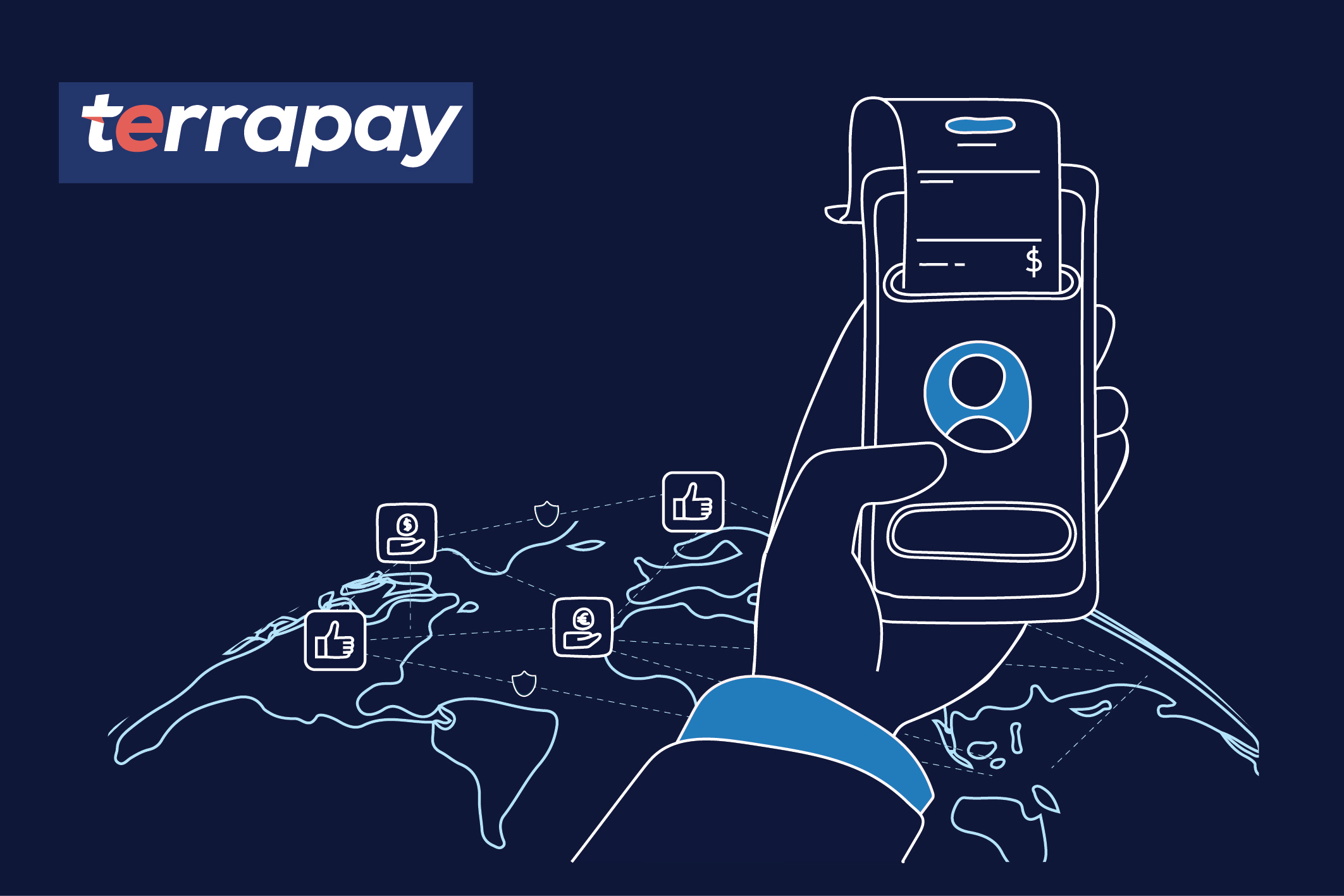
Streamlined data insights and agile data preparation for Terrapay
Learn how Terrapay, a leading cross-border payment infrastructure solution provider built the Terrapay Intelligence Platform (TIP) with Enrich to achieve operational efficiency through use cases such as Forecasting, Partner Performance Analytics, Customer Journey Analytics, and more.
Read More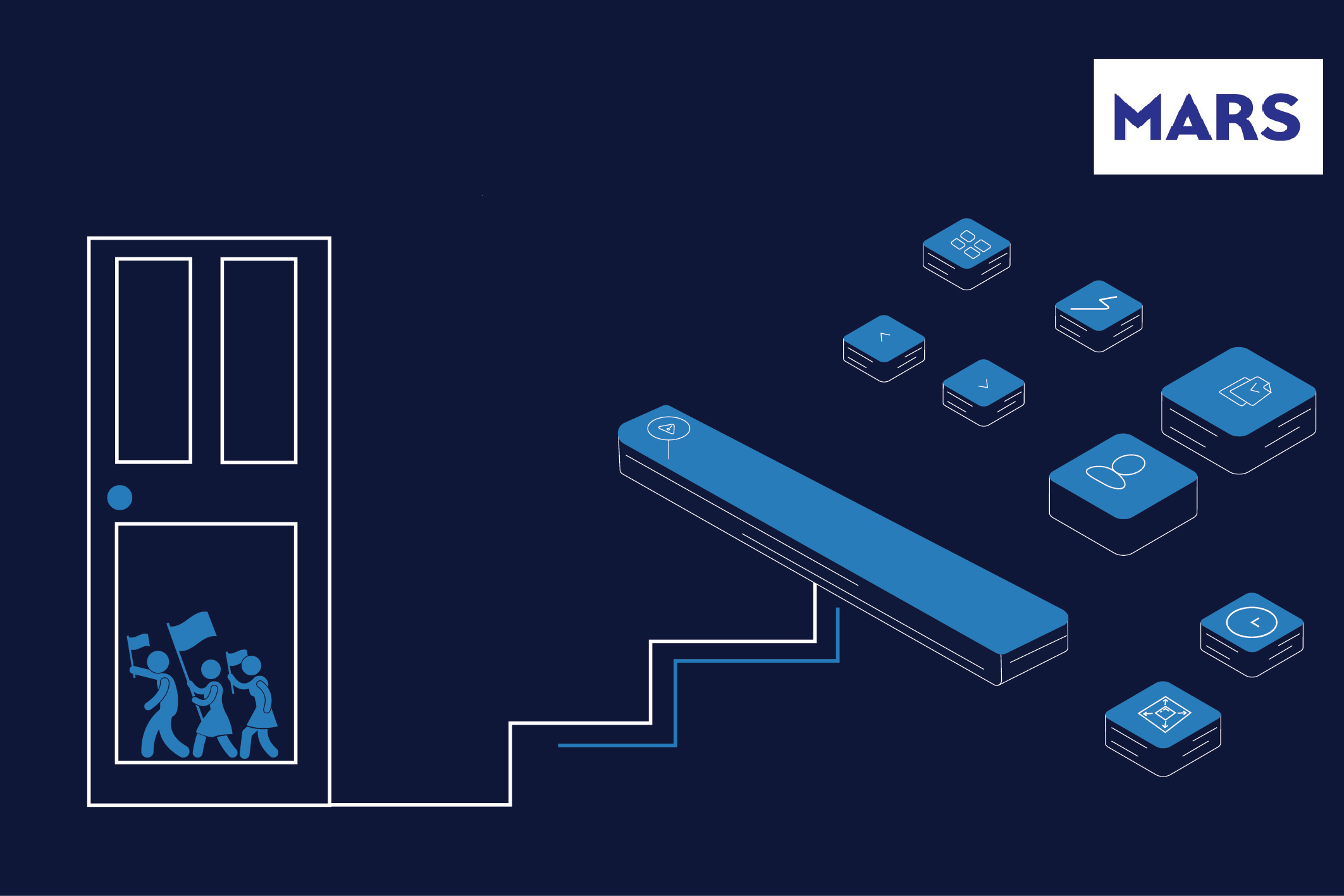
How Mars Took Steps to Evaluate the Potential Impact of the “Great Resignation”
Learn how Mars, a Fortune 100 CPG company collaborated with Scribble Data to assign a “probability of attrition” through data, and ML modeling.
Read More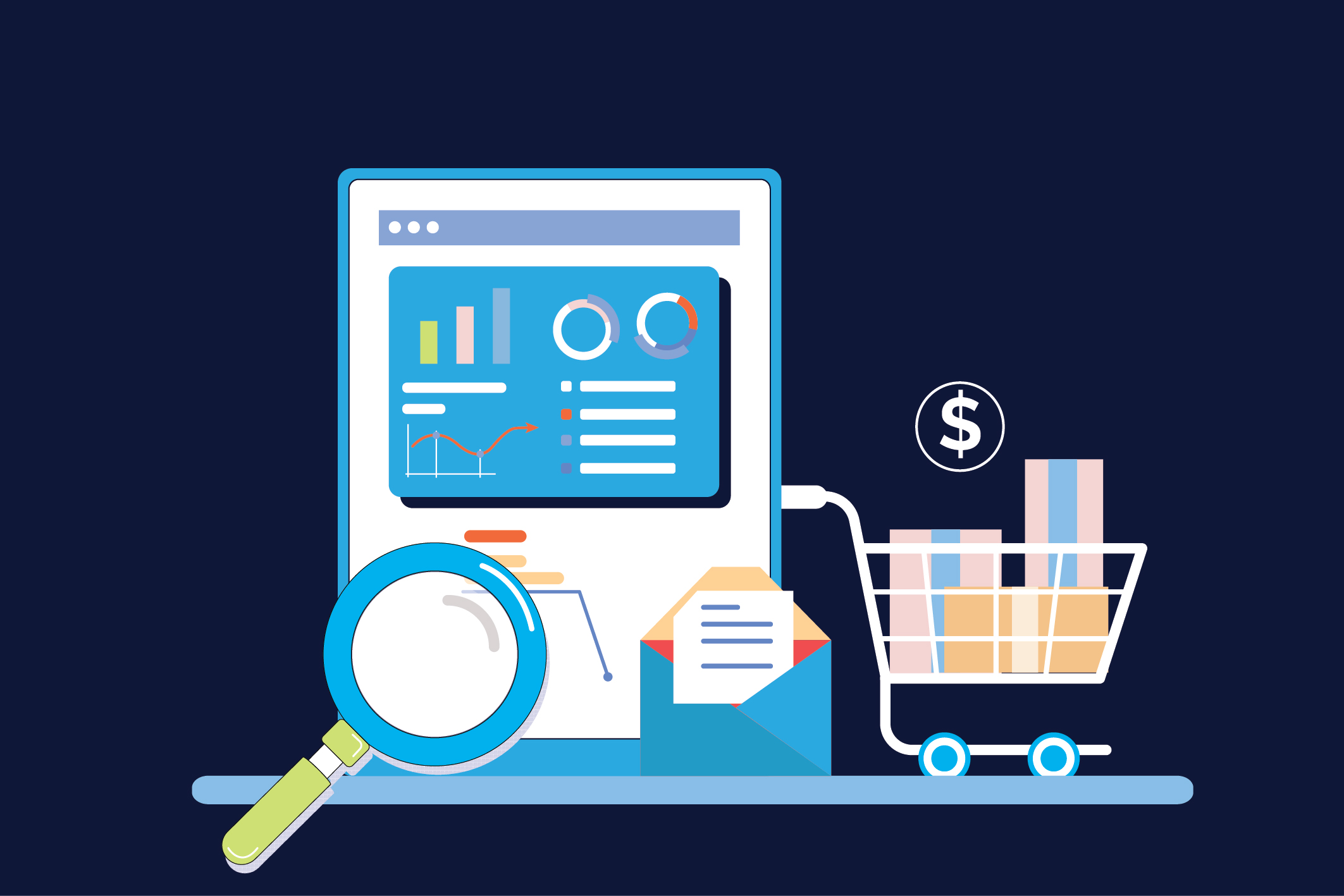
Accelerated ML Engineering for a Leading E-Commerce Brand
Learn how a leading e-commerce brand selling children’s apparel built their data intelligence platform on Scribble Data that supported the rapid development and deployment of use cases such as Product Listing Optimization and Re-ordering.
Read More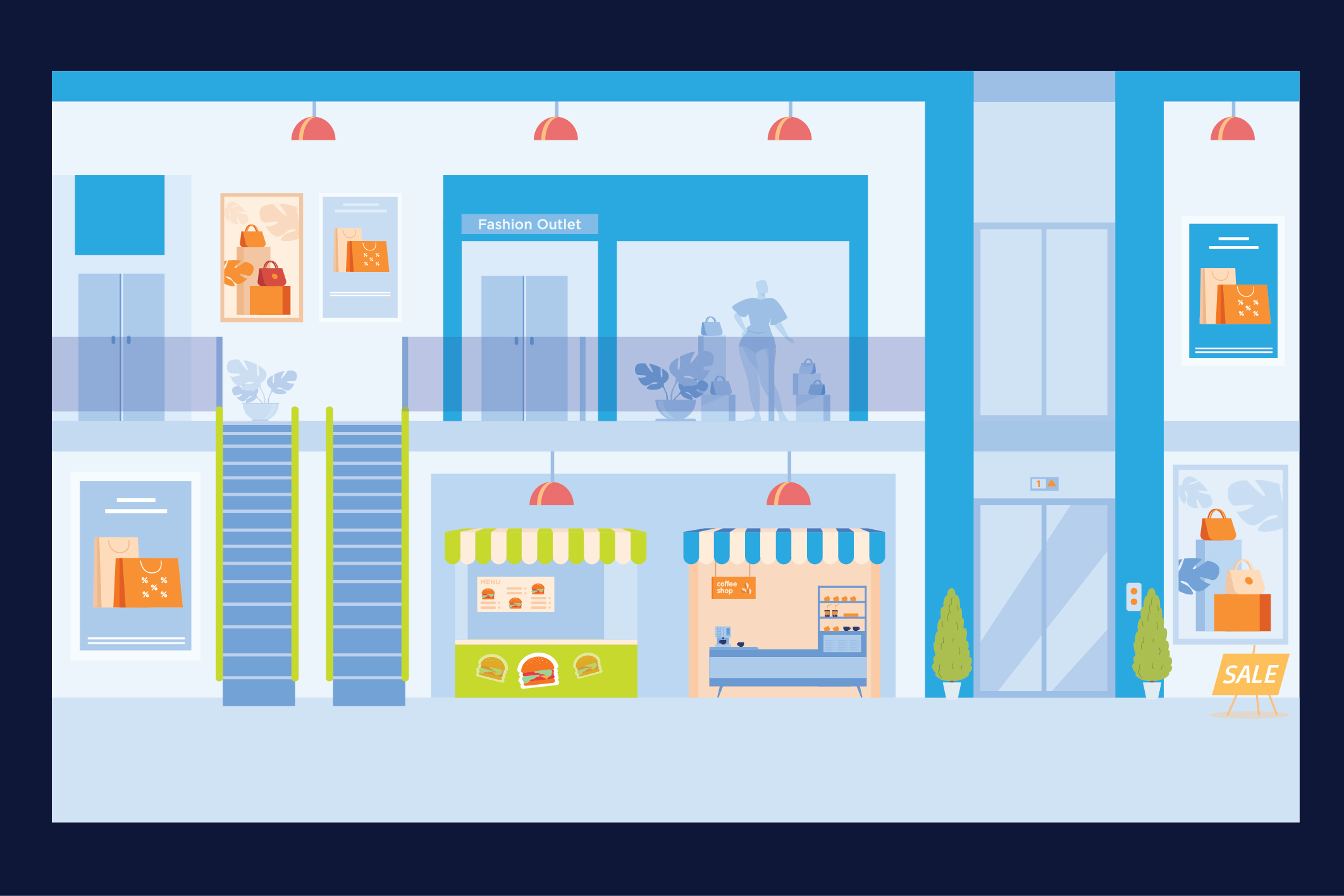
Understanding Shopping Paths at a National Mall Chain
Learn how a nationwide mall chain used Scribble Data’s Enrich platform to identify patterns of shopper footfalls, determine the timing and location of ads, and achieve a significant M-o-M increase in revenue.
Read More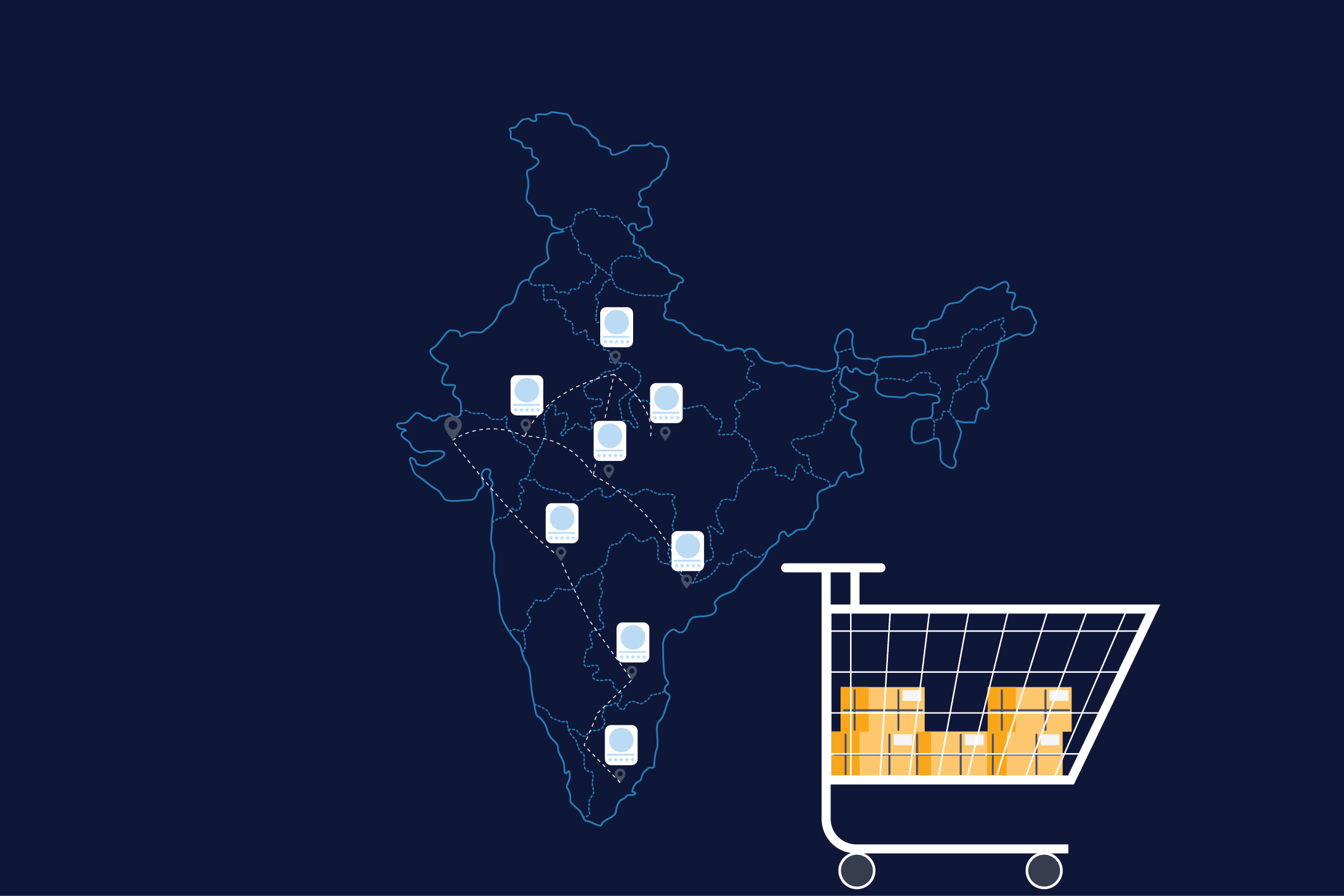
A National Level Retail Store Chain
A national level retail chain in India leverages Scribble Data Enrich for developing an accurate understanding of their buyer personas, their distribution, demand and context at a fine granularity to address multiple operational use cases.
Read MoreVideos
MLOps Conference: Fast Development of ML Applications
Malavika Lakireddy (VP, Product Development, Zeotap), Saransh Verma (Director-Analytics, TerraPay), and Dr. Venkata Pingali (Co-Founder and CEO of Scribble Data) talk about the challenges in developing ML applications.
Watch NowOrchestrating AI Assistants at the Enterprise
Dr. Venkata Pingali, CEO of Scribble Data, talks about the complexities of orchestrating multiple AI systems to work seamlessly together.
Watch NowLifecycle of a Data Product with Dr. Venkata Pingali
Watch this session where Dr. Venkata Pingali, Founder & CEO of Scribble data shares his perspective on Data Products, the types of Data Products, and the lifecycle of Data Products with the Data Heroes community.
Watch NowCustomer Testimonial: Cloudphysician
Dileep Raman, Cloudphysician’s Co-founder and Chief of Healthcare, talks about how Scribble Data enabled them to rapidly build pipelines to transform their data and get daily updated feature sets as well as trustworthy models – all in less than 4 weeks!
Watch NowCustomer Testimonial: Mars, Inc.
Dr. Vidyotham Reddi of Mars, Incorporated–a leading US-based multinational CPG manufacturer of confectionery, pet food, and other food products and a provider of animal care services, talks about his experience of working with Scribble Data. Learn how his team at Mars was able to assign a “probability of attrition” to employees, calculated based on which […]
Watch NowWhat’s the deal with sentient AI? – Achint Thomas
Sentience in AI has always been the holy grail for computer science. What qualifies as AI sentience, and what is just another case of a model mimicking the data it’s trained on?
Watch NowAnatomy of a production ML feature engineering platform – Venkata Pingali
This talk draws upon the Scribble’s experience in building and evolving a production feature engineering platform, and the many conversations we have had with user data scientists. The talk will focus on the learnings, and not on the Scribble product itself, and expand on the talk from Fifth Elephant Mumbai in Jan 2019 on reducing […]
Watch NowAccelerating ML using Production Feature Engineering Platform by Venkata Pingali
Anecdotally, only 2% of the models developed are productionized, i.e., used day to day to improve business outcomes. Part of the reason is the high cost and complexity of productionization of models. It is estimated to be anywhere from 40 to 80% of the overall work.
Watch NowGlobal Feature Store Meetup #13 – Scribble Data
Feature stores have been traditionally designed for complex ML applications (Big-ML) that normally assume clear and high value propositions, long lead times, skilled staff, and advanced methods. Sub-ML is a space of mid-complexity ML applications where there is higher uncertainty in terms of value, methods used, available staffing, and speed is critical. Sub-ML is interesting […]
Watch Now