The Fintech market is valued at $110.57 billion in 2020 and will reach $698.48 billion by 2030. It is one of the fastest-growing industries with a CAGR of 20.3%. Fintech companies faced a surge in demand as customer practices and banking habits changed during the COVID-19 era. The industry overall saw an increase in user adoption. For instance, the real-time share of global electronic transactions in 2020, during COVID, was 70.3 bn, which was a whopping 41% more than 50 bn in 2019.
With this unprecedented rapid growth, Fintech companies need to harness their data faster to unearth key business insights that will help them make faster business decisions. Apart from worrying about privacy and security compliances across geographies, Fintech companies need to expand their offerings according to regions, figure out what works and what doesn’t, and provide users with a personalized experience.
But as they scale and the need for business-critical information increases. By just using AI and ML tools, Fintech companies aren’t able to leverage the goldmine of data they have at their disposal effectively. This could be due to various reasons including lack of expertise, maintenance issues, or regulation issues for cross border transactions.
With the help of right data science practices that focus on driving outcomes, Fintech companies can shorten their time taken to build an in house decision-making product that helps them solve persistent business problems in just a couple of weeks, as opposed to 6-8 months!
But before we dive into the Postmodern Data Stack, let’s see how data science as we know has evolved.
Evolution from Conventional to Postmodern Data Stack
The Modern Data Stack is a compilation of tools and methods that help organizations handle and use big data. Organizations today use PyTorch, Apache, Kafka, Snowflake, BI tools and more to make their data workflows easier. As the scale increases, the requirement to collect and prepare data, build ML models and deploy models involves an end-to-end machine learning workflow and has to be equipped with big-data tools.
As the data inflow increases, there is also a rapid increase in the adoption of these tools for each stage of the data lifecycle. It becomes challenging for organizations to handle as the requirement at every point during scale keeps changing. The current data stack can get pretty overwhelming – you need an army of resources – from data scientists to ML and data engineers, DevOps, software engineers, and more. These engineers need to continuously keep learning to adapt to the rapid changes in what we’ve come to know as the modern data stack (MDS), in addition to just doing their regular job. This causes burnout and attrition amongst available talent.
As an organization, a large data team can also do more harm than good as multiple users are performing multiple operations. If you’re from a small-mid sized enterprise, many of the data tools might not make sense for you at your scale.
With the current MDS there are longer feedback loops. ML initiatives can take upto a year to build and decipher. If you’re a small-mid sized organization it becomes challenging as you don’t know if you’re on the right path, decision-making becomes slower during the hyper growth phase. This is one of the reasons why ML models fail 90% of the time.
As data science keeps scaling with new technology and operational methods, people are expected to keep learning. Sometimes this can result in clashing results and ambiguous views on how to approach business issues, thereby delaying crucial business decisions. According to Gartner, only 53% of the projects make it from prototype to production, despite organizations spending a lot to maximize their ROI on ML. Many organizations wait upto months when the decision could’ve been taken in just mere weeks.
As the way we work evolves, our data warehouses need to adapt to the change.The combination of the right technology mix and solution focus makes it possible to align product value to use cases with the correct TTV mix, which helps in achieving 5x faster time to value. This is where the postmodern data stack or ML at reasonable scale can play a key role.
It uses three major principles to process data that can be utilized by companies :
Data Extraction
Data extraction is done through two processes ELT and ETL – Extract, Load, Transform and Extract, Transform and load. This process helps gather all the siloed data through ingestion. The raw data streams are gathered from the organization’s data libraries and cleaned up before they’re inserted into a data store.
Data Storage
The data storage is the place where refined data can be stored and accessed by teams. These are cloud-based warehouses where information can be fetched using the preferred intelligence tool used by organizations.
Data Transformation
The data stored can now be used to get meaningful insights. There are two approaches that you can typically consider depending on the size and complexity of your data – Machine Learning (ML) and Business Intelligence (BI). Today, we see BI and ML coexist across data-driven organizations. However, while both of these functions focus on providing actionable inputs for decision-making, their implementation complexity, design, and end consumers are very different.
- BI tools can only handle a limited amount of complexity and size of datasets. Their scope is limited when it comes to providing solutions to use cases with increasing degrees of complexity, primarily focused on decision making
- ML engineers and data scientists are expected to solve the challenge of decision-making. But, due to the lack of bandwidth (and unavailability of data science talent, not to mention the lack of prepared datasets taking up over 80% of the time), these use cases are often put on the backburner.
A significant set of problem statements and use cases that fall into the no-man’s land between BI and ML (due to the lack of the data science team’s bandwidth and being outside the capabilities of traditional BI tools), presents an opportunity for immediate impact and rapid scaling for fast moving businesses. Examples of such use cases include benchmarking, segmentation, classification, and risk assessment. This is where the postmodern data stack, or what we consider a part of Sub-ML comes in.
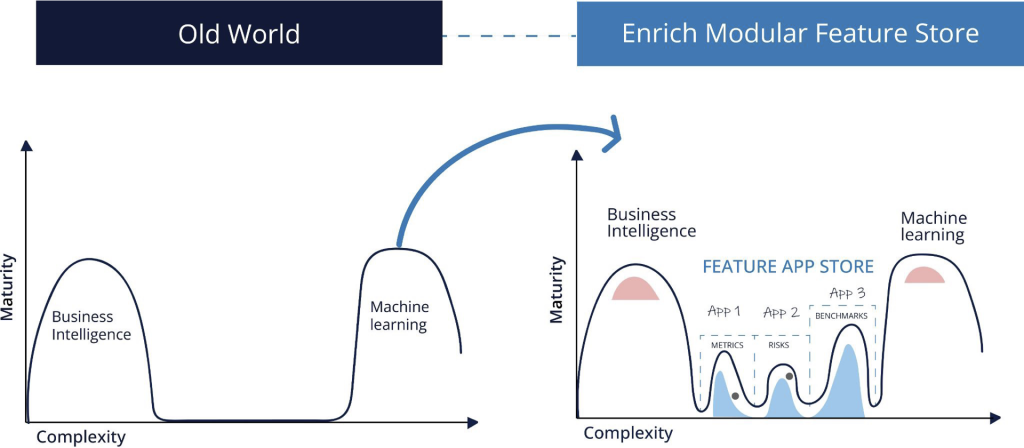
The evolution of data-driven use cases
How the Postmodern Data Stack enables emerging Fintech data trends
Fintech technology market is segmented based on three areas – deployment-based, application-based, and end-user based. By deployment, it is divided into on-premise and cloud, by technology it is split into API, AI, blockchain, RPA, and data analytics, and by end-user, it is differentiated into banking, insurance, securities, and more.
These Fintech institutions implement advanced technologies to provide integrated and value-added solutions and are trying to expand rapidly. To make operations agile here’s how the Postmodern Data Stack comes into play:
Digital and Online Banking
Online banking is a trend that has been witnessed in recent years. Since its advent, people have reduced their time going to banks for mundane day-to-day tasks. Digital banks form the backbone for a better financial technology future- using them consumers can have access to financial information and data at their fingertips.
Even though we see a surge in daily users, banks still struggle to uphold their digital presence. Constant data security threats loom over the horizon as digital vulnerabilities are more discreet and can cast a lasting impact on users and their data. It’s not just the profiles at risk but also their personal data.
Anomaly Detection
When you use traditional systems you have a bunch of messy data which needs to be coordinated with different processes and technologies. This usually took time and by the time the information reached you – it was already too late! Using the postmodern data stack, you can eliminate risks and fraud even before they happen. When millions of transactions are happening simultaneously you can quickly spot transactions that increase risk and complete your root-cause analyses faster than before. This helps you prevent money laundering, cyber security threats, credit risks, other anomalies, and unlawful behaviors you might detect.
Data Extraction and Reconciliation
For every Fintech company data, extraction is an important step. This process enables companies to take better control of their finances and help manage their resources more efficiently. Data extraction helps Fintech organizations maximize their value and potential so that they can drive quicker processes and resolve risks or anomalies earlier. They need information such as logs, complex transformations, and other datasets at their fingertips. Beyond just extracting data efficiently, they also need to reconcile data from various sources to ensure data fidelity. This will enable them to compare and resolve differences between multiple datasets.
But the current challenge is that extracting relevant data from the plethora of data streams available internally is messy and time intensive. The scarcity of talent has made this job harder for organizations.
With the postmodern data stack, fintech organizations don’t need to rely on ad-hoc scripts and pipelines to their datasets. A single server deployment with zero DevOps and pre-integrated building blocks can speed up the dev and deployment, making Ops efficient. All this can be done in a matter of minutes and saves Fintech companies to have one less worry – what do we do about our data?
Metrics
Metrics are key to any Fintech organization. It shows important data like business health, performance, risks, and conversion data. Companies still use the old method of relying on BI tools for monitoring their metrics. The traditional dashboard that is generated has to go through the following steps; requirement clarification, data sourcing, pipeline implementation, dashboard creation, and user acceptance testing. This method of dashboard generation requires back and forth between the IT teams and various business teams to give their sign-off. The process is tedious and time-consuming.
In the postmodern data stack, a metric store is available so that the end-to-end delivery with the commonly monitored metrics is readily available and ready to ship. This reduces turn-around-time and unnecessary back and forth with teams so that ML teams can concentrate on creating custom, complex, metrics that aren’t already defined in the metrics store instead of implementing and maintaining straightforward metrics for the entire organization.
Benchmarking and Forecasting
Oftentimes, data is siloed within teams making it difficult to quickly arrive at business competency decisions. This leads to communication inefficiencies and institutional knowledge being embedded in opaque ways across teams and specific individuals.
Postmodern Data stack effectively battles this issue – it ensures that all the data is stored in a single place so that teams have ready access to everything they need in order to make quick decisions. For Fintech companies, this is a blessing because as they scale they need to compare and benchmark the performance metrics of their different partners, routes and regions. This requires end-to-end monitoring from payment gateways to banks to financial institutions and more. In a traditional method, this information gets siloed within various teams and the time taken to secure the data is longer. Therefore, by the time the decision is made, it would already impact a certain amount of revenue.
Benchmarking insights can be used to derive which payment gateway provider is important for the EU or APAC and which one for the US. For example, if provider X is really good with great transaction completion rates but isn’t that well-performing in the EU compared to provider Y, the business can take a crucial business decision on who to partner with in the EU or US. This benchmarking data can be extended to various offerings enabling quick decisions and getting the right insights to drive better results while scaling.
Personalized Experiences
Personalized services have become table stakes for all industries. To stand out from the competition and gain customer attention, businesses need to offer them a personalized experience. In Fintech, personalization means interacting with the user at the right time and using the right channel to solve their needs.
With traditional data systems, this can be quite a challenge, as millions of users are transacting using the platform on a regular basis. Customization and personalization can be overwhelming and confusing with the data presented. To overcome this challenge, Fintech companies should use customer profiling to have precise customer data and insights at their disposal.
Companies need to gauge user behavior to provide them with a sticky experience. With the post-modern data stack, Fintech organizations can finally make sense of their data by diving into their marketing and user data, and identifying the high-value customer segments and the product affinities. By doing this,organizations can open up a plethora of up-sell and cross-sell solutions that can unlock additional gates of revenue.
AI and ML
AI and ML are known for the data that they can provide us. This data will enable banks to automate a whole range of processes and manage transactions effectively. But it isn’t as easy as it seems.
In the banking industry, integrating technology into the tech stack and having access to actionable data is still a challenge. To enable AI and big data you need to teach AI through ML. You need large amounts of data to train your system – but the current issue is that there is a lack of high quality, clean and annotated data.
Fintech challenges that the Postmodern Data Stack can help overcome
Helps companies see through data
Many organizations can be wrangled with the amount of information they have that cannot be made sense of. For Fintech companies making sense of the data is the need of the hour. It is a goldmine that can unearth business secrets and challenges.
Using Scribble’s Enrich platform, Fintech organizations can now differentiate and make sense of their complex data sets to make real-time decisions.
Security and Privacy
Enrich helps proactively combat risks before they even happen. Fintech companies can now monitor their data and prevent cyber threats, find risky user behavior, and detect anomalies and other concerns before they become huge.
Data-Driven Decisions
With Enrich, Fintech companies can make decisions that can unlock the next phase of growth. The output is trustworthy, auditable, and reproducible – this can be used to analyze different areas of their business and make the process more intelligent.
If you want to know how Enrich can impact your organization’s metrics, here’s how Terrapay did it! With data-backed insights, they were able to actively resolve problems, and go from concept to go live within just 3-4 weeks instead of 6-8 months! They were able to concentrate on their customer journeys and increase business revenue through upsells and cross-sells.
By eliminating data silos you offer more value to your organization, customer, and stakeholders Compared to traditional ML models, with sub-ML use cases we are already seeing 5-8x faster time to value. Enrich helps accelerate your response time, predict changes and prepare for scenarios. It helps your team to make faster, confident, real-time, data-backed decisions that reduce the margin of error – thereby propelling your business forward.