The Oakland Coliseum was abuzz, the air thick with anticipation. In the dimly lit back office, Billy Beane, the General Manager of the Oakland Athletics, sat hunched over a cluttered desk. Papers were strewn everywhere, but Billy’s focus was on a single sheet filled with numbers, statistics, and player names.
The Athletics, with one of the smallest budgets in Major League Baseball, were up against Goliaths, teams with deep pockets and star-studded line-ups. But Billy had an ace up his sleeve, a secret weapon that none of the big teams had even considered.
Paul DePodesta, his young assistant, walked in, laptop in hand. “The numbers don’t lie, Billy,” he said, his voice filled with a mix of excitement and trepidation.
They weren’t just looking at batting averages or home runs. They were delving deep, analyzing on-base percentages, and other overlooked statistics. They were challenging the very fabric of baseball wisdom.
As the season progressed, the Athletics began to defy expectations. Players that other teams had written off were performing at peak levels. The team was winning, not through big names or hefty contracts, but through data. They had turned raw statistics into a winning strategy – Moneyball – a tangible product that delivered results on the field.
Much like Billy Beane’s revolutionary approach in baseball, businesses today are faced with a deluge of data. The challenge? Turning that raw data into actionable insights, into products that can guide decisions and strategies. This transformation, this art of crafting data into invaluable products, is what we delve into next. Welcome to the world of data products.
What is a Data Product?
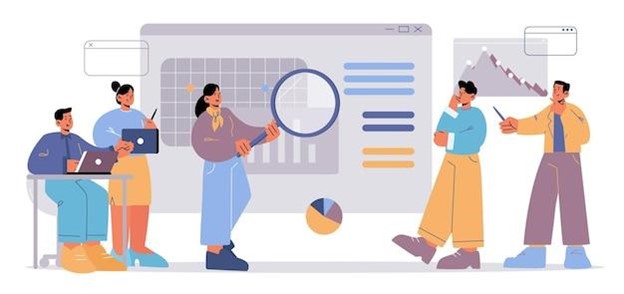
Source: freepik
In today’s digital landscape, data is omnipresent, but its true value lies not in its abundance but in its application. Just as raw statistics in baseball can be transformed into a winning strategy, raw data in business can be molded into products that drive decisions, predict trends, and offer actionable insights. This is the essence of data products.
Gone are the days when static reports and charts sufficed. Businesses today require solutions that cater to specific needs, offering real-time insights tailored to evolving scenarios. Data products rise to this challenge, providing context-rich, interactive, and actionable insights.
Some of the key characteristics of data products include:
- Discoverability: Ensuring users can easily find and access the data product.
- Interoperability: Its ability to integrate seamlessly with other systems.
- Ownership: Clear accountability for the data product’s maintenance and updates.
- Continuous Management: Regular updates and improvements based on user feedback and changing data landscapes.
Data products excel in transforming raw data into actionable business strategies. They are designed to be user-friendly, adaptable to changing business needs, and are continuously refined to ensure they remain relevant and effective.
The Data Product Canvas: Your Blueprint for Success
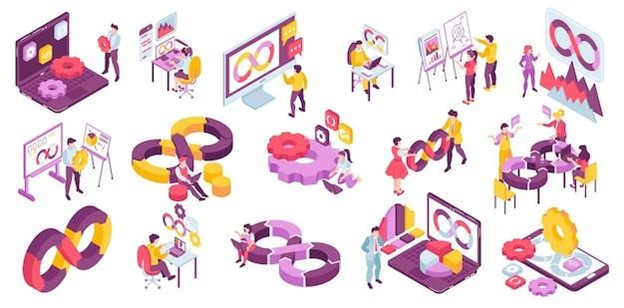
Source: freepik
The journey from raw data to actionable insights can often feel like navigating a dense forest without a map. Enter the Data Product Canvas (DPC) – your compass in this intricate landscape. Just as an architect wouldn’t dream of constructing a building without a detailed blueprint, data professionals shouldn’t embark on the creation of a data product without the guiding structure of the DPC.
A Foundational Tool for Data Product Development
The DPC is not just another framework; it’s a holistic tool designed to bridge the gap between technical intricacies and business objectives. By offering a structured approach to data product development, the DPC ensures that all stakeholders, from data scientists to business leaders, are aligned in their vision and objectives. It’s the canvas on which the masterpiece of your data product will be painted.
Components of the Data Product Canvas
The DPC is divided into several components, each addressing a critical aspect of data product development:
- Problem Statement: Before diving into data, it’s crucial to have a clear understanding of the problem you’re aiming to solve. This section ensures that the data product addresses a genuine business need.
- Data: What data sources are available? How reliable are they? This component delves into the raw material of your product – the data itself.
- Users: Who will be using the data product? Understanding the end-users’ needs, preferences, and technical capabilities is paramount to creating a product that truly adds value.
- Business Value: At the end of the day, a data product must deliver tangible business benefits, be it increased revenue, cost savings, or improved customer satisfaction. This section ensures that the product aligns with broader business goals.
- Metrics: How will success be measured? Defining clear, quantifiable metrics is essential to evaluate the effectiveness of the data product.
- Functional Requirements: This dives into the technical side of things, outlining the features and capabilities the data product must possess.
- Non-functional Requirements: Beyond the core functionalities, considerations like scalability, security, and performance come into play.
- Constraints: Every project has its limitations, be it budget, time, or resources. Outlining these early on ensures realistic planning and execution.
- Stakeholders: Identifying everyone involved in the project, from decision-makers to end-users, ensures clear communication and alignment throughout the development process.
The beauty of the DPC lies in its ability to foster collaboration. By providing a clear, visual representation of the data product’s objectives and requirements, it ensures that both technical and non-technical stakeholders have a shared understanding. No more miscommunications or misaligned goals; with the DPC, everyone is on the same page.
As we delve deeper into the world of data products, keep the DPC in mind. It’s the lens through which we’ll explore the nuances of understanding data consumers’ needs, packaging data products, and building effective solutions.
Why Every Data Product Development Starts With An Understanding of The User
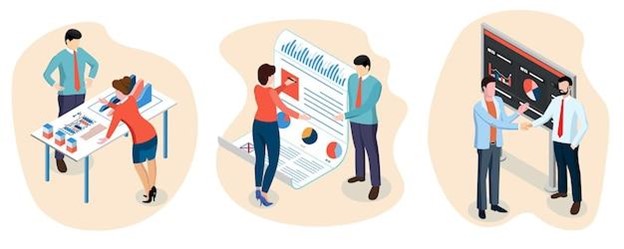
Source: freepik
When building data products, one principle stands paramount: know your user. While the technical intricacies and architectural nuances are vital, at the heart of every successful data product lies a deep understanding of its end-users.
- Diverse Data Consumers: The world of data consumers is as varied as it is vast. From data scientists delving deep into analytics to business analysts seeking actionable insights, each user approaches a data product with unique expectations and objectives. Recognizing this diversity is the first step in crafting a product that truly caters to its audience.
- Technical Abilities: Not all data consumers are created equal, at least not in terms of technical prowess. While a data scientist might revel in the complexities of raw data, a marketing executive might seek a more streamlined, visual representation. Designing a data product means striking a balance, ensuring it’s robust enough for the tech-savvy while remaining accessible to those less versed in data intricacies.
- Decision-Making Process: Data products aren’t just tools; they’re decision-making aids. Whether it’s a financial analyst forecasting market trends or a supply chain manager optimizing logistics, understanding how users harness data products to make decisions is crucial. This insight informs the design, ensuring the product provides the right information in the right format at the right time.
- Combining Data Products: In today’s interconnected digital landscape, it’s not uncommon for users to combine insights from multiple data products. A sales manager might integrate sales data with market research, crafting a comprehensive view of the business landscape. Anticipating such integrations and designing data products that can seamlessly interoperate is a hallmark of forward-thinking data product architecture.
- Feedback Loop: The journey of a data product doesn’t end at launch. In fact, that’s just the beginning. Establishing a continuous feedback loop with users ensures the product evolves, adapts, and improves over time. It’s this iterative process, this commitment to refinement, that transforms a good data product into a great one.
Understanding the consumer is the compass that points the way, ensuring that our data products resonate, inspire, and empower their intended users.
Packaging a Data Product
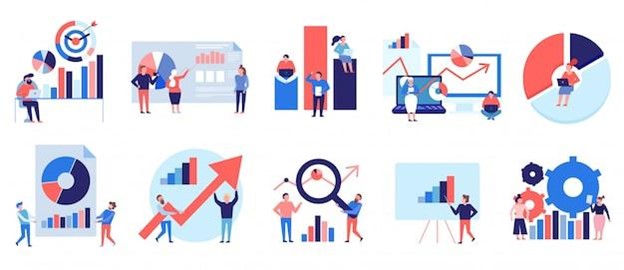
Source: freepik
In the bustling marketplace of data products, presentation is paramount. Just as a beautifully wrapped gift entices the recipient, a well-packaged data product captivates its users, making the complex appear simple and the overwhelming seem manageable.
- Metadata: Imagine diving into a novel without a title, author’s name, or even chapter headings. Confusing, right? Metadata serves as the “book jacket” of a data product, offering users a snapshot of what to expect. It provides context, ensuring users understand the source, nature, and relevance of the data they’re exploring.
- Documentation: A data product, no matter how advanced, is only as good as its user manual. Comprehensive documentation demystifies functionalities, guiding users through features and ensuring they harness the full potential of the product. It’s the roadmap that navigates users through the data landscape.
- Tools for Analysis: Data, in its raw form, can be daunting. By equipping users with analytical tools—be it visualization dashboards, filtering options, or predictive analytics capabilities—a data product transforms from a mere repository of information to a dynamic platform for insights.
- Optimal Access Methods: In our digital age, flexibility is key. Whether a user prefers a desktop interface, a mobile app, or an API integration, ensuring varied and optimal access methods enhances user experience, making data interaction seamless and intuitive.
- Training and Support: Even the most intuitive data products can pose challenges. By offering training sessions, tutorials, and dedicated support channels, businesses ensure users aren’t left in the lurch. It’s about empowering users, ensuring they feel confident and competent in their data interactions.
- Security Protocols: In a world where data breaches make headlines, security isn’t just a feature; it’s a necessity. Implementing robust security protocols, from encryption to access controls, safeguards not just the data but also the trust users place in the product.
Packaging a data product is an art and a science. It’s about aesthetics and functionality, presentation, and utility. How we present data is just as crucial as the data itself. After all, in the words of Leonardo da Vinci, “Simplicity is the ultimate sophistication.”
Building Effective Data Products
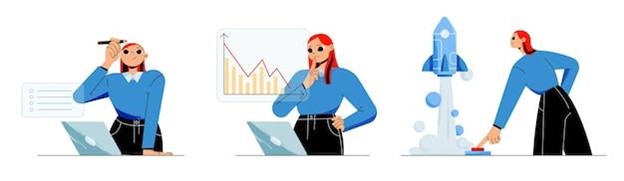
Source: freepik
Crafting a data product that is effective is no small feat. Let’s discuss the art and science of building truly effective data products.
- Identifying Pain Points: Every great invention starts with a problem. Before diving into design and development, it’s crucial to understand the challenges the data product aims to address. Is it about improving customer retention? Identifying risks? Enhancing supply chain efficiency? By pinpointing the pain points, we set a clear direction for the product’s journey.
- Defining the Target Audience: A data product designed for data scientists will differ vastly from one intended for marketing professionals. Recognizing the primary users and understanding their technical proficiency, needs, and preferences ensures the product is tailored to resonate and provide value.
- Building a Cross-Functional Team: Data products are multifaceted, and so should be the team behind them. A blend of data engineers, data scientists, UX designers, and business strategists ensures a holistic approach, where technology meets user experience, and data meets actionable insights.
- Developing a Launch Plan: A grand entrance matters! Strategizing the rollout, from beta testing to full-scale launch, ensures users are onboarded smoothly. It’s about setting expectations, offering training, and ensuring the product integrates seamlessly into the users’ workflow.
- Iterative Testing: Perfection is a journey, not a destination. Continuous feedback loops, beta testing, and refinements based on user feedback ensure the product evolves, adapts, and remains relevant in the ever-changing data landscape.
Consider a retail giant using a data product to predict customer churn, tailoring marketing campaigns based on individual preferences. Or a healthcare provider leveraging data to optimize patient outcomes and streamline operations. These are but glimpses of how effective data products drive transformation across industries.
Building an effective data product is iterative, collaborative, and, above all, user-centric. It’s not just about the data; it’s about the impact it creates.
Data Products and Machine Learning
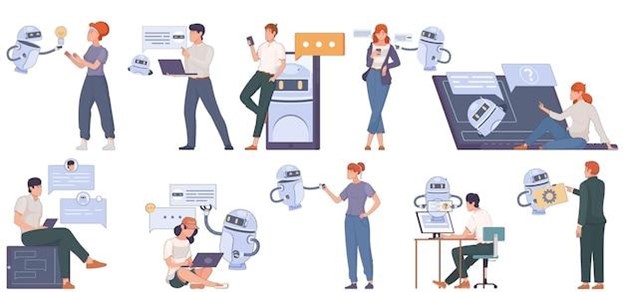
Source: freepik
In the world of data products, machine learning (ML) is a critical component, transforming raw data into predictive insights and actionable recommendations. While data products provide the structure and interface, machine learning fuels the intelligence behind these products, enabling them to adapt, learn, and improve over time.
Machine learning, at its core, is about training algorithms on vast amounts of data to make predictions or decisions without being explicitly programmed for that task. When integrated into data products, ML algorithms can sift through massive datasets, identify patterns, and generate insights that would be impossible or highly time-consuming for humans to derive.
Popular Data Products Powered by Machine Learning:
- Recommendation Systems: Platforms like Netflix and Spotify use machine learning to analyze user behavior and preferences, offering personalized movie or music recommendations. These systems continuously learn from user interactions, refining their suggestions over time.
- Search Engines: Google’s search engine, arguably one of the most influential data products, relies heavily on machine learning to rank pages and provide users with relevant search results. The algorithms assess user intent, the relevance of web pages, and the quality of content to deliver the best possible results.
- Voice Assistants: Siri, Alexa, and Google Assistant are prime examples of data products that utilize natural language processing, a subset of machine learning, to understand and respond to user queries.
- E-commerce Personalization: Amazon’s product recommendations are driven by machine learning algorithms that analyze a user’s purchase history, viewed items, and other users’ behaviors to suggest products that the user might be interested in purchasing.
The development of ML-driven data products is inherently iterative. As more users engage with the product, more data is generated, which can then be fed back into the system to train the algorithms further. This continuous cycle of feedback and refinement ensures that the data product remains relevant and improves its accuracy and effectiveness over time.
Feedback loops are crucial in this context. They allow for the continuous collection of user interactions and feedback, which can be used to adjust and refine the machine learning models. For instance, if a recommendation system consistently suggests products that users don’t engage with, this feedback can be used to adjust the model and improve future recommendations.
Machine learning amplifies the capabilities of data products, enabling them to provide dynamic, personalized, and continuously improving solutions. As businesses increasingly rely on data-driven decision-making, the fusion of data products and machine learning will be at the forefront of this revolution.
Challenges and Considerations in Data Product Development
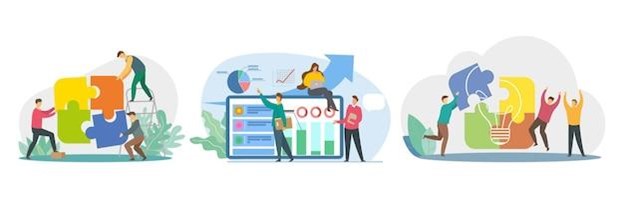
Source: freepik
The journey of developing a data product, while promising in its potential, is not without its hurdles. As with any technological endeavor, there are challenges to navigate and considerations to keep in mind to ensure the product’s success and relevance.
- Continuous Management and Updates: Data is dynamic. What’s relevant today might not be tomorrow. As businesses evolve, so do their data needs. A data product must be continuously managed, updated, and refined to cater to these changing requirements. This involves not just updating the data but also refining algorithms, incorporating new features, and ensuring the product aligns with the current business strategy.
- Scalability Issues: As organizations grow, so does the volume of their data. Handling this increased load without compromising on performance is a significant challenge. Strategies like data mesh or data fabric come into play here. A data mesh, for instance, decentralizes data ownership and architecture, allowing individual teams to take ownership of their domain-specific data, ensuring scalability and agility.
- Data Quality and Consistency: Garbage in, garbage out. The insights a data product provides are only as good as the data it’s built upon. Ensuring data quality, consistency, and accuracy is paramount. This involves regular data audits, validation checks, and cleaning processes.
- Ethical Considerations: With great power comes great responsibility. Data products, especially those driven by machine learning, can inadvertently introduce biases or make decisions that have ethical implications. It’s crucial to ensure that data products are transparent in their workings and that ethical considerations are at the forefront of their development.
- User Feedback and Iterative Refinement: The end-users are the best judges of a data product’s effectiveness. Regularly collecting user feedback and incorporating it into the product’s refinement is essential. This iterative approach ensures that the product remains aligned with user needs and continues to deliver value.
In the process of building data products, challenges are a given. But with a proactive approach, continuous refinement, and a keen focus on delivering value, these challenges can be transformed into opportunities for growth and innovation.
Conclusion: The Future of Data Products
As we look to the future, the landscape of data products is only set to evolve further. With advancements in AI, machine learning, and data science, the potential applications and capabilities of data products will expand, offering even more nuanced and tailored solutions to business challenges. Industries, from healthcare to finance to retail, will find new avenues to leverage these products, creating a ripple effect of innovation and growth.
Yet, as with any journey, the path to successful data product development is one of learning and adaptation. For organizations looking to embark on this journey, the mantra is clear: start small, iterate often, and always keep the end-user in focus. By doing so, they can ensure that their data products are not just technologically sound but also resonate with the needs and expectations of their users and pave the way for a future where data-driven decision-making is the norm, not the exception.